To effectively manage data, comply with legal regulations, and improve data-related business processes in every company, including the pharmaceutical industry, it is necessary to introduce data modeling standards. These standards bring the rules for storing, organizing, and accessing data, which is becoming increasingly important in the face of growing data complexity and regulatory requirements. The result is a solid data model that serves as a blueprint for designing and managing data structures. It ensures data consistency, reliability, and ease of access.
Importance of data modeling standards
Implementing data modeling standards is important for several reasons. Firstly, data should consistently ensure uniformity across various systems, databases, and departments, facilitating seamless data integration and communication.
Pharmaceutical data, like any other, can have invalid values, differing measurement units, or missing attributes necessary for proper processing. Therefore, data teams responsible for data quality should understand these limitations before deciding on the conditions for using or not using the data. This is where it helps to have modeling standards that ensure standardization and high quality of data, manifested in increased accuracy, uniformity, and reliability.
From a business perspective, this is vital for faster decision-making and regulatory compliance. For instance, promoting drugs to doctors and hospitals in the USA requires adherence to the Sunshine Act, mandating the reporting of payments made to individuals or institutions.
Another challenge is a large volume of data received, which must be collected, exchanged, and processed to provide holistic insights into business operations. A single standardized data model (example in Figure 1B) supports this by reducing data redundancy, as fewer data records need to be loaded into the data warehouse.
Additionally, data modeling standards facilitate collaboration across different departments of the enterprise by using the same data naming convention and following approved procedures. A shared understanding of the data model enables collaborative and informed business decisions based on consistent and accurate data while engaging a broader audience.
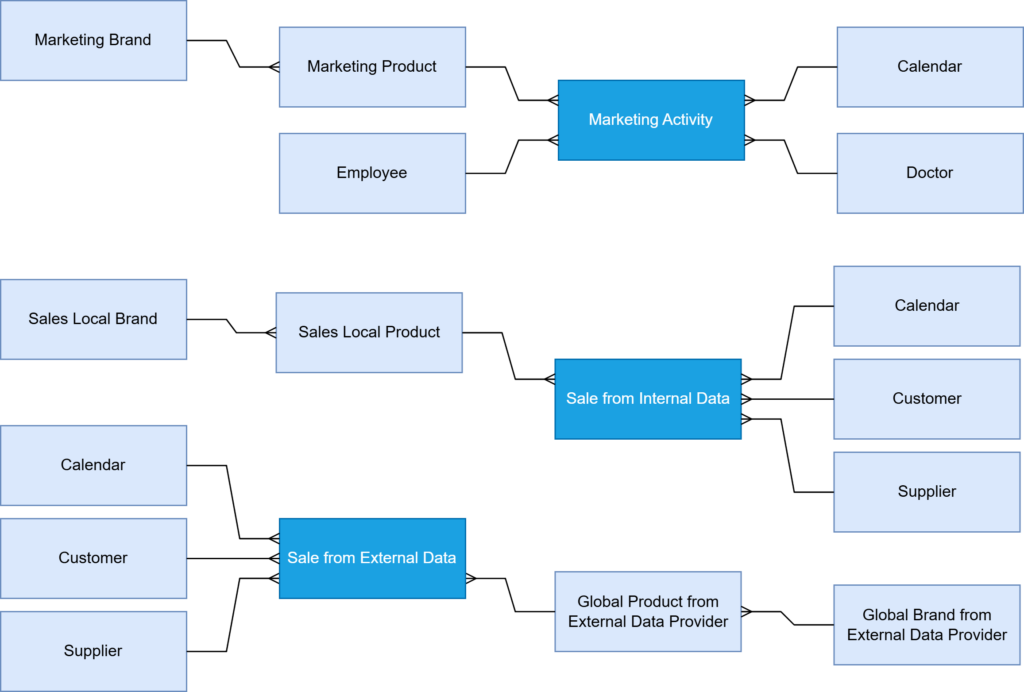
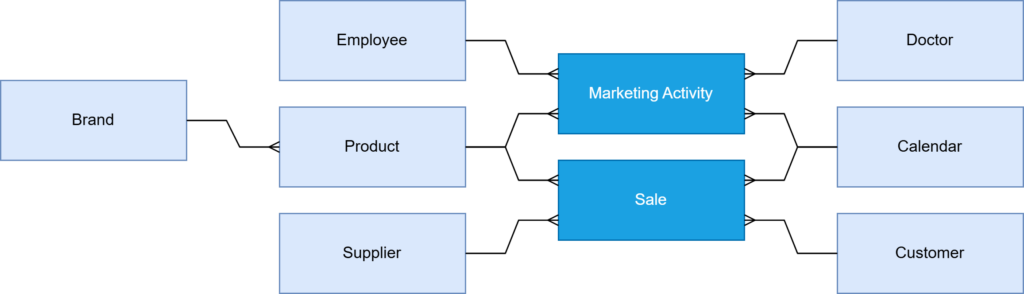
Identification of key challenges in pharmaceutical data modeling
Pharmaceutical companies frequently encounter multiple challenges in implementing effective data modeling standards. These challenges start with the data itself, its quantity and complex structure, then their combination into one consistent data set, all while being limited by available resources and legal regulations.
One significant issue is the complexity of managing and integrating vast and diverse data sets from numerous sources and by different development teams. Reaching a consensus on objects that contain the same domain data stored across multiple systems, especially product data, is frequently difficult. Poor information governance around master data exacerbates organizational complexity, and a high degree of overlap in master data, such as customer data stored across various objects in the enterprise data model, is common.
Development carried out by independent teams, both internal and vendors’, forces coordination between them while expanding and optimizing the structure and content of the enterprise data warehouse. They must share the common knowledge of the data model, data modeling standards, and architectural principles. Changes introduced by them should be in accordance with approved procedures and documented in the same location and format.
Organizations often face significant challenges with data quality issues. These include master data such as customer and product information, as well as data from legacy systems with varying standards. The absence of a comprehensive MDM (Master Data Management) that defines different levels of data sources (primary and secondary) makes integration and expansion of the data model more difficult.
We encountered such challenges with one of our clients. To effectively address them, we assembled a team that included data management experts and consultants to help design and implement a robust data model and standards across the entire pharmaceutical company. In the next chapter, we describe how we coped with these challenges and why an appropriate approach to the data model strongly affects the quality and availability of business analytics.
Harmonizing brand and product data in a pharmaceutical company
A pharmaceutical company, one of BitPeak’s clients, needed to optimize an MDM system specifically for brand and product names by consolidating objects containing the same domain data from various sources. This initiative aimed to create a unified brand and product data hierarchy, standardize naming conventions, and integrate data from multiple database systems.
After preliminary workshops and a thorough pre-analysis conducted by BitPeak’s Business-System Analysts, the project focused on several key areas: data harmonization, content consolidation, updating and optimizing the MDM, and incorporating process automation wherever feasible to tailor the solution to client.
The process began with identifying the data sources that should be covered by the MDM. The client’s enterprise operates on a data model based on several different types of data sources (Figure 2):
- Sales CRM — containing both actual and historical sales transactions at a product level, offering a comprehensive view of sales performance.
- Marketing CRM — with data covering aspects of the employee’s marketing activities, including promotional efforts, campaigns, and customer engagement.
- Master Data — providing business objects that hold the most critical and universally accepted information, i.e. data dictionaries and hierarchies, shared across the organization, serving as the single source of truth.
- Flat files — with additional information required by the business, often used for ad-hoc reporting, or supplementary data that is not available in structured databases.
- Launch Planning CRM — for collecting data on the process before introducing products to the market, including timelines, projected quantities, and anticipated sales.
- External Data Provider — with sales data gathered by the global pharmaceutical data company, providing external benchmarks and insights to validate internally collected data.
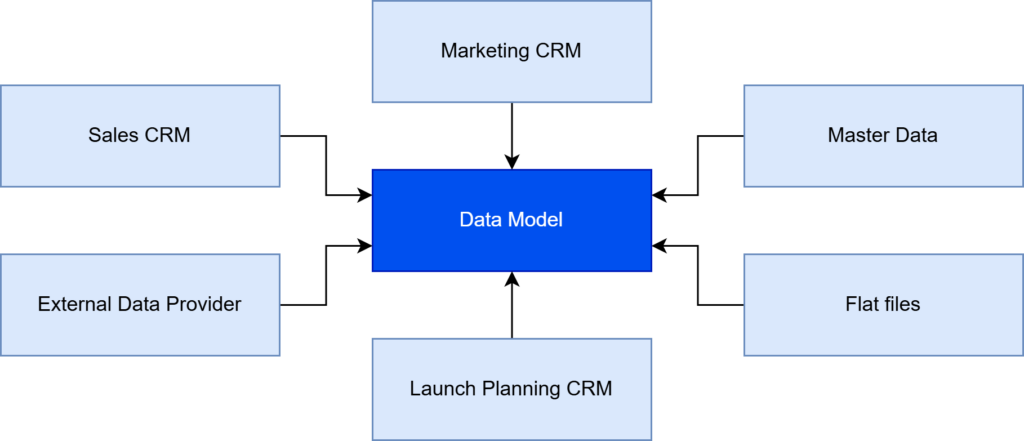
Data objects and specific fields requiring standardization were then selected. In our case, we focused on brand and product naming across the company’s diverse database systems. The client’s company departments were using incompatible global and local names (Figure 1A), making it much more difficult for data analysts to link information from various business areas (e.g., sales, marketing) and, consequently, track products at different stages of their life cycle in the market. Due to existing data inconsistencies, analyzing the data was challenging.
Designing the data model was the next crucial step. The Business-System Analyst, in collaboration with a Data Architect, developed a structured hierarchy for brand and product names (Figure 1B). This involved creating unified definitions and naming standards to ensure consistency across all platforms and compliance. The Development Team then prepared the environment for integrating data from various systems, migrating this data into a central master data repository.
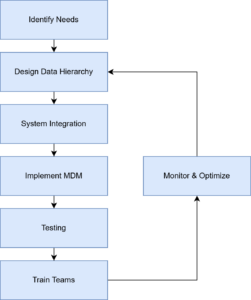
Implementing changes in the MDM system required careful configuration according to the developed data hierarchy. The Development Team conducted rigorous integration and data consistency tests to ensure that the system functioned correctly, and that data integrity was maintained. Following successful integration, training sessions were organized for the marketing and sales teams, demonstrating how to use the MDM system and highlighting the benefits of having a unified database. Monitoring and optimization are continuous processes. Support kept track of the MDM system’s performance and collected feedback from users. They are then forwarded to an analyst for in-depth analysis and collection of business requirements. Any necessary adjustments and optimizations were implemented by the Development Team to enhance the system efficiency and user experience.
The project successfully delivered a unified data hierarchy for brand and product names. By gathering data in one consistent repository, it became more accessible and reliable, significantly improving the consistency and quality of marketing and sales analyses. Moreover, thanks to standardized brand and product names around the world, business awareness and knowledge about market performance on a global and local scale have increased. The reports obtained on their basis enable cross-interpretation of harmonized data sets from various data systems and getting a holistic image of the pharmaceutical company’s operations from the pre-launch process, through product implementation, marketing, to performance monitoring. This leads to making the right and more conscious decisions regarding the international pharmaceutical business.
Summary
At BitPeak, we always prioritize proactive cooperation with our clients, guided by the highest business value of the proposed data solutions. Therefore, we pay attention to effective data management and compliance with data modeling standards, which constitute the foundation for the good operation of any global company. By using recognized best practices and modern technologies, companies can increase the accuracy, consistency, accessibility, and regulatory compliance of their data. The result is better business intelligence that enables clients to make more informed decisions, contributing to gaining a competitive edge on the market.