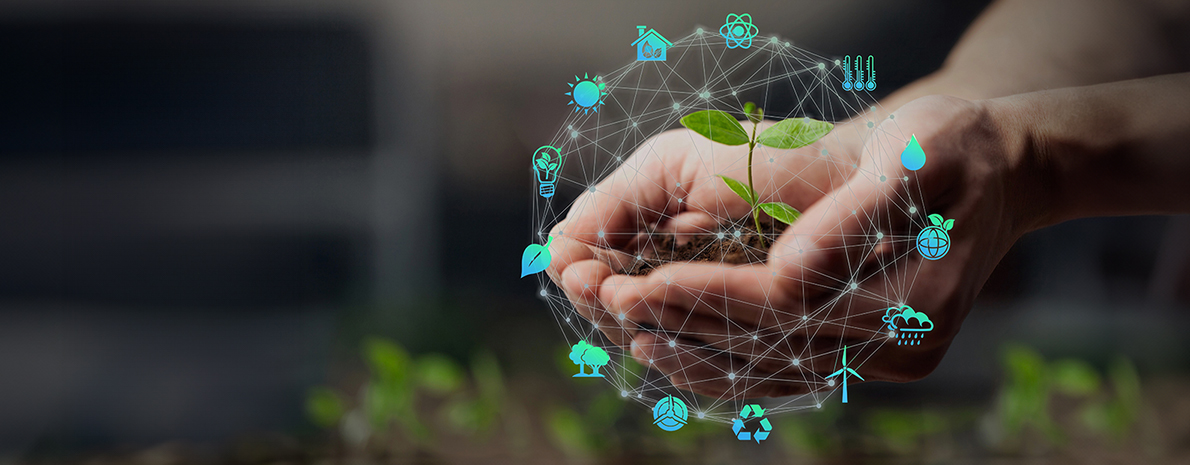
Data&ESG - Part 2: Power BI Dashboard
Take a Peak and learn a Bit about creating effective and easy-to-use Power BI dashboard for your ESG reporting needs!
Read more
Take a Peak and learn a Bit about creating effective and easy-to-use Power BI dashboard for your ESG reporting needs!
Read moreThe newest Databricks compute method comparison by our Solution Architect Champion
Read moreIn recent years Environmental, Social, and Governance (ESG) went from a secondary concern or bullet point on a CSR leaflet to a key part of corporate strategies. Why?
There are many reasons from research indicating that sustainability is good for a company’s long-term success to legal obligations, criteria for cheaper financing, and better employee relations. Aligning business and ESG strategies is both a challenge to overcome and an opportunity to seize.
Fortunately, both of which can be simplified thanks to advanced data gathering, analytics, and reporting tools, which allow companies to monitor their supply chains, forecast ESG risks, and keep up with new regulations. In our series of articles, we will guide you through the whole process
Before we go further, let us make sure we are all on the same page, by answering the basic question. What exactly does ESG mean?
E stands for the environmental criteria, which consider how a company performs when preserving and mitigating the harm to the natural environment. This can mean:
S stands for the social criteria, which assess a company’s capacity and performance in managing relationships with communities around it, employees, shareholders, or, simply put – stakeholders. For example:
G represents the Governance criteria, which revolve around the rules, practices, and processes by which a company is directed and controlled. Governance in the ESG context focuses on how a company ensures that its operations are transparent, compliant, and aligned with the interests of its shareholders and other stakeholders. This involves:
Now we know what ESG is. But why should you care about it? Aside from ethical reasons.
The present relevance of ESG is underpinned by its integration into investment decisions, corporate strategies, and regulatory frameworks during the last few years.
For many bigger companies, those who are included in increasingly numerous sustainability regulations, ESG compliance is no longer optional, though for now, it is mostly focused on reporting and prevention of greenwashing, such as SFRD and CSDR. Even if they are omitted, they probably want to work with those who are or obtain financing from ESG-focused funds. Especially since some of the biggest financial management funds, such as Blackrock, steer increasingly in the ESG direction.
However, the question of the permanence of such direction persists as recently some moved away from sustainability-focused funds.
Sustainability factors can influence investor preferences, government grants, consumer behavior, and financing possibilities. Because of that ESG-compliant financial assets are projected to exceed $50 trillion by 2025, accounting for more than a third of the projected $140.5 trillion in global assets under management. This significant growth, from $35 trillion in 2020, reflects the increasing mainstreaming of ESG criteria into the financial sector and beyond.
The research also indicates that ESG funds outperform their less sustainable counterparts over both shorter and longer periods of time.
It is also worth noting that there is no “Too big for ESG”. Tech giants such as Amazon, Google, and Apple, have faced scrutiny regarding their ESG practices, especially in the social and governance aspects. This not only made them eat bad PR but also motivated regulators to take a closer look at them.
This means that the growth of ESG assets and the increasing integration of ESG criteria into business practices reflect a paradigm shift in the business and investment landscape. And as sustainable governance becomes more critical, companies are urged to adopt comprehensive strategies to meet new, evolving standards, and ensure that their operations align. Well, and report everything about that alphabet soups such as CSRD, or SFDR or CSDDD requires them to.
Knowing all that, let us ask about what new challenges will appear and how to make ESG strategy an asset rather than bothersome new obligations. We will start by identifying business and legal challenges and risks.
First, let’s take on business challenges, which consist mostly of strategic and operational risks. For example, poor corporate governance can weaken risk management in ESG areas and across different parts of the business, leaving companies open to major strategy mistakes and operational problems, such as misalignments, missed investments and internal conflicts. As ESG regulations get more complex and wide-reaching, companies need a comprehensive strategy that embeds ESG governance throughout their operations. This approach helps ensure everyone is on the same page and reduces the risks of disjointed ESG efforts.
Then we have dangers to reputation. The impact of failing to address ESG issues can be considerable. Almost half of investors are willing to divest from companies that do not take sufficient ESG actions, highlighting non-compliance’s reputational and financial risks. Well, at least half of the investors self-report that way. Additionally, consumers are more willing to buy products from companies without ethical standards, while employees stay longer in companies that care for their well-being!
Secondly, we have financing opportunities.
As we established earlier, investors are often more willing to invest in sustainable companies. But that is not all when it comes to ESG and financing.
Many companies now favor green bonds or ESG-linked loans to fund projects that are good for the environment, getting a better deal terms such as higher principal or lower interest rates thanks to high demand from investors who want sustainable options.
Additionally, governments and regulatory groups are getting involved too, offering grants, subsidies, and incentives to push companies towards sustainable practices. This financial aid makes it more appealing and financially feasible for companies to pour money into green projects and social efforts. On top of that, sustainable investment funds are funnelling money into companies known for their solid ESG practices, providing an often cheaper alternative to the usual financing methods.
Lastly, we have the carbon credits market, which gives companies a financial incentive to cut emissions, letting them sell any surplus credits or balance out their own emissions, effectively paying them for being eco-friendly. It is also worth noting that regulatory incentives and partnerships between the public and private sectors often include ESG objectives, nudging companies to take on public benefit projects while sharing the financial and operational load.
Many legal regulations focus on ESG criteria. So, we will point them out to underscore their scope without an in-depth look. The third article in the series will focus on legal obligations and ways to manage them more easily.
After outlining the future challenges, we can talk about things that we at BitPeak specialize in! That means solving problems with data. But let’s talk specifics, how can we use new technologies, AI, Data Engineering and Visualizations to make future more sustainable?
The most essential things regarding sustainability initiatives and regulatory ESG compliance are accurate reporting and information management. Usually, the process can be complex due to the multitude of different standards and high complexity of operations in enterprise-scale companies. However, it can be made easier with environmental management information systems, which can aid in accurately reporting greenhouse gases, compliance reporting, and tracking product waste from generation to disposition.
To illustrate, we can look at projects utilizing no-code platforms for ESG data collection and reporting. Its goal is to address the challenges of fragmented and geographically dispersed data for ESG compliance, which many companies, especially those with many global branches, struggle with. By developing a workflow-management tool that automates communication with data providers, digitizes data collection, and centralizes tracking and approval statuses, operational risks and errors can be significantly reduced while the reporting cycle is shortened. Read more here.
The need for robust, auditable ESG data has never been more critical, especially with the SEC’s proposed climate disclosure rules. Organizations are moving beyond static Excel spreadsheets, utilizing real-time ESG data management software to manage compliance obligations and beyond. This approach facilitates compliance and delivers higher business value, sustaining a competitive advantage by investing in ESG initiatives. Sounds interesting?
Think about the possibilities with dynamic and scalable dashboards that quickly show you what areas you are ahead and where you lag behind the demands of regulators and investors. Take a look at our showcase of GRI compliant ESG report right here as a perfect example of this approach: BitPeak ESG Intelligence tool
AI-based applications offer new ways to enhance ESG data and risk management. For example, ESG risk management solutions use machine learning to streamline regulatory compliance management. They analyze complex requirements and produce structured documents highlightning key elements organizations need to meet their obligations, thereby facilitating compliance.
Especially with the advent of recent solutions based on the idea of RAG (Retrieval Augmentation Generation) and semantic knowledge bases! Being able to always easily access just the right information from internal sources or insights about coming regulation with one question to a specialized and fully secure language model is simply an implementation issue.
Data science techniques, specifically predictive analytics, can also be used to identify and mitigate sustainability risks before they become problematic and harder to mitigate. Firms can predict potential vulnerabilities using data models that incorporate various indicators such as historical financials, ESG performance metrics, and even social media sentiment.
For example, Moody’s Analytics ESG Score Predictor employs a proprietary model to estimate ESG scores and carbon footprint metrics, providing insights for both public and private entities across a multitude of sectors.
But reporting and predicting, while important, is not the be-all and end-all. So let’s take a look at how the integration of IoT and advanced data analytics can be used to reduce environmental footprint. IoT sensors deployed across various segments of operations, from manufacturing floors to logistics, gather real-time data on energy use, waste production, and resource consumption. This data is then analyzed to pinpoint inefficiencies and adjust processes accordingly, leading to significant reductions in energy consumption, waste, and overall environmental impact.
A fine example of this is BitPeak’s project during which we cooperated with SiTA to optimize fuel usage in air travel as well as SAF (sustainable aviation fuel) logistics, while ensuring compliance with EU SAF targets! Another practical application of this approach can be seen in smart manufacturing facilities where IoT sensors control and optimize energy use, substantially lowering operational costs and reducing carbon emissions.
As you can see there are a lot of ways and tools to not only make ESG compliance easier, but also more profitable, which is the key to the green future. As regulations and market trends continue to move towards the sustainability, leveraging new tech will be key to maintaining both integrity and competitive advantage in the business landscape.
So, what’s now? We have discussed what ESG is, why you should be interested in it and ways in which data can help you with legal compliance and aligning your business and sustainability strategy. We discussed ESG’s growing importance due to stakeholder, and financer demands for sustainable business practices and identified challenges in ESG compliance, including strategic, operational, and legal hurdles.
We highlighted IT solutions like our GRI compliant report or AI which analyzes ESG performanceand helps you meet ESG criteria. In the end we want you to leave knowing that leveraging data technology is crucial for businesses to navigate the complexities of ESG compliance efficiently. But that is not all! We also plan to write an article exploring the way to design and implement optimal and efficient Power BI dashboard to deal with your ESG and sustainability reporting needs! Look forward to it appearing on our blog soon!
Take a Peak and learn a bit about ESG, reasons why you should care about it, and how technology can help you keep up with the challenges of sustainability!
Read more
In the process of building RAGs (Retrieval Augmented Generation), chunking is one of the initial stages, and it significantly influences the future performance of the entire system. The appropriate selection of a chunking method can greatly improve the quality of the RAG. There are many chunking methods available, which were described in the previous article. In this one, I focus on comparing them using metrics offered by LlamaIndex and visualizing chunks created by individual algorithms on diverse test texts.
The LlamaIndex metrics are used to compare RAGs constructed based on chunks generated by various chunking methods, and the chunks themselves will also be compared in various aspects. Additionally, I propose a new chunking method that addresses the issues of currently available chunking methods.
Conventional chunking methods sometimes create chunks in a way that leads to loss of context. For instance, they might split a sentence in half or separate two text fragments that should belong together within a single chunk. This can result in fragmented information and hinder the understanding of the overall message.
Currently available semantic chunking methods encounter obstacles that the present implementation cannot overcome. The main challenge lies in segments that are not semantically similar to the surrounding text but are highly relevant to it. Texts containing mathematical formulas, code/algorithm blocks, or quotes are often erroneously chunked due to the presence of these elements in the text, as the embeddings of these fragments are significantly different.
Classical semantic chunking typically results in the creation of several chunks (including usually several very short ones, such as individual mathematical formulas) instead of one larger chunk that would better describe the given fragment. This occurs because the currently created chunk will be „terminated” when it encounters the first fragment that is semantically different from chunk’s content.
The issues described above led to the development of the chunking algorithm called “semantic double-pass merging”. Its first part resembles classical semantic chunking (based on mathematical measures such as percentile/standard deviation). What sets it apart is an additional second pass that allows merging of previously created chunks into larger and hence more content-rich chunks. During the second pass, the algorithm looks „ahead” two chunks. If the examined chunk has sufficient cosine similarity with the second next chunk it sees, it will merge all three chunks (the current chunk and the two following ones), even if the similarity between the examined chunk and the next one is low (it could be textually dissimilar but still semantically relevant). This is particularly useful when the text contains mathematical formulas, code/algorithm block, or quotes that may „confuse” the classical semantic chunking algorithm, which only checks similarities between neighboring sentences.
The first part (and the first pass) of the algorithm is a classical semantic chunking method: perform the following steps until there are no more sentences available:
To address scenarios where individual sentences, such as quotations or mathematical formulas embedded within coherent text, pose challenges during semantic chunking, a secondary pass of semantic chunking is conducted.
If the cosine similarity from the fifth step exceeds the merging threshold, it indicates that the middle-examined chunk was a „snippet” (possibly a quote/mathematical formula/pseudocode) with different embedding values from its surroundings, but still a semantically significant part of the text. This transition ensures that the resulting chunks will be semantically similar and will not be interrupted at inappropriate points, thus preventing information loss.
Thresholds in the algorithm control the grouping of sentences into chunks (in the first pass) and chunks into larger chunks (in the second pass). Here’s a brief overview of the three thresholds:
For optimal performance, set the appending_threshold and merging_threshold relatively high to ensure cohesive and relevant chunks, while keeping the initial_threshold slightly lower to capture a broader range of semantic relationships. Adjust these thresholds based on text characteristics and desired chunking outcomes. Additionally, examples should be added: monothematic text should have higher merging_threshold and appending_threshold in order to differentiate chunks, even if the text is highly related, and to avoid classifying the entire text as a single chunk.
The comparative analysis of key chunking methods were conducted in the following environment:
For the purpose of comparing chunking algorithms, we used LangChain’s SpacyTextSplitter for token-based chunking and sent_tokenize function provided by nltk for sentence-based chunking. After using sent_tokenize, the chunks were created by grouping them according to a predetermined number of sentences. The proposition-based chunking was performed using various OpenAI GPT language models. For semantic chunking with percentile breakpoint LangChain implementation was used.
The first test involved assessing how specific models perform (or not) with a simple example where topic change is very distinct. However, the description of each of the three topics consisted of a different number of sentences. Parameters for both token-based chunking and sentence-based chunking were set so that the first topic is correctly classified.
To conduct the test, the following methods along with their respective parameters were used:
Both token-based and sentence-based chunking encounter the same issue: they fail to detect when the text changes its topic. This can be detrimental for RAGs when „mixed” chunks arise, containing information about completely different topics but connected because these pieces of information happened to occur one after the other. This may lead to erroneous responses generated by the RAG.
The above image excellently illustrates why clustering methods should not be used for chunking. This method loses the order of information. It’s evident here that information from different topics intertwines within different chunks, causing the RAG using this chunking method to contain false information, consequently leading to erroneous responses. This method is definitely discouraged.
Typical semantic chunking struggles to perfectly segment the given example. Various values of the breakpoint parameter were tried, yet none achieved perfect chunking.
The primary goal of the first pass of the double-pass algorithm is to accurately identify differences between topics and only connect the most obvious sentences together. In the above visualization, it is evident that no mini-chunk contains information from different topics.
The second pass of the double-pass algorithm correctly combines previously formed mini-chunks into final chunks that represent individual topics. As seen in the above example, the double-pass merging algorithm handled this simple example exceptionally well.
The next test was to examine how a text containing pseudocode would be divided. The embeddings of pseudocode snippets would significantly differ from the embeddings of text snippets that cut through them. Ultimately, the text and its description should be combined into one chunk to maintain coherence. For this purpose, a fragment of text from Wikipedia about the Euclidean algorithm was chosen. In this comparison, the focus was on juxtaposing semantic chunking methods, namely classical semantic chunking, double-pass, and propositions-based chunking:
Semantic chunking using percentiles was unable to comprehend the text as a single chunk. The entirety of the sample text was merged into one chunk only when the breakpoint value was set to the maximum value of 100 (which merges all sentences into one chunk).
Semantic chunking using percentiles with a breakpoint set to 60, which allows for distinguishing between sentences on different topics, struggles with this example. It cuts the algorithm in the middle of a step, resulting in chunks containing fragments of information.
The double-pass merging algorithm performed admirably, interpreting the entire text as a thematically coherent chunk.
The proposition-based chunking method first creates a list of short sentences describing simple facts and then constructs specific chunks from them. In this case, the method successfully created one chunk, correctly identifying that the topic is uniform.
To assess how different chunking methods perform on longer text, the well-known 'PaulGrahamEssayDataset’ available through LlamaIndex was utilized. Subsequently, simple RAGs were constructed based on the created chunks. Their performance was evaluated using the RagEvaluatorPack provided by LlamaIndex. For each RAG, the following metrics were calculated based on 44 questions provided by LlamaIndex datasets:
More detailed definitions of faithfulness and relevancy metrics are described in this article.
To conduct this test, the following models were created:
For comparison purposes, the average time and costs of creating chunks (embeddings and LLM cost) were juxtaposed. The obtained chunks themselves were also compared. Their average length in characters and tokens was checked. Additionally, the total number of tokens obtained after tokenizing all chunks was counted. The cl100k_base tokenizer was used to calculate the total token count and the average number of tokens per chunk.
Chunking method | Average chunking duration | Average chunk length [characters] |
Average chunk length [tokens] |
Total token count | Chunking cost [USD] |
Token-based | 0.08 sec | 458 | 101 | 16 561 | <0.01 |
Sentence-based | 0.02 sec | 395 | 88 | 16 562 | 0 |
Semantic percentile-based | 8.3 sec | 284 | 63 | 16 571 | <0.01 |
Semantic double-pass merging | 16.7 sec | 479 | 106 | 16 558 | 0 |
Proposition-based using gpt-3.5-turbo | 9 min 58 sec | 65 | 14 | 2 457 | 0.29 |
Proposition-based using gpt-4-turbo | 1 h 43 min 30 sec | 409 | 85 | 6 647 | 17.8 |
Proposition-based using gpt-4 | 40 min 38 sec | 548 | 117 | 5 987 | 29.33 |
As we can see, classical chunking methods operate significantly faster than methods attempting to detect semantic differences. This is, of course, due to the higher computational complexity of semantic chunking algorithms. When looking at chunk length, we should focus on comparing two semantic methods used in the comparison. Both token-based and sentence-based methods have rigid settings regarding the length of created chunks, so comparing their results in terms of chunk length won’t be very useful. Chunks created by classical semantic chunking using percentiles are significantly shorter (both in terms of the number of characters and the number of tokens) than chunks created by semantic double-pass merging chunking.
In this test, no maximum chunk length was set in the double-pass merging algorithm. As a result of tokenization on the created chunks, the sum of tokens in each tested approach turned out to be very similar (except for the proportion-based approach). It’s worth noting the chunks generated by the proposition-based method. The use of the gpt-4 and gpt-4-turbo models results in a significantly longer process time for a single document. As a result of this extended process, the longest chunks are created, but there are relatively few of them in terms of the total number of tokens. This occurs because this approach compresses information by strictly focusing on facts. On the other hand, the propositions-based approach based on gpt-3.5 generates significantly fewer propositions, which then need to be stitched together into complete chunks. As a result, the execution time is much shorter.
The differences in the time required for proposition-based chunking with various models stem from the number of propositions generated by each model. gpt-3.5-turbo created 238 propositions, gpt-4-turbo created 444, and gpt-4 created 361. Propositions generated by gpt-3.5-turbo were also simpler and contained individual facts from multiple domains, making it harder to combine them into coherent chunks, hence the lower average chunk length. Propositions generated by gpt-4-turbo and gpt-4 were more specific and numerous, facilitating the creation of semantically cohesive chunks.
When comparing costs, it’s worth emphasizing that the text used for testing various methods consisted of 75 042 characters. Creating chunks for such a text is possible for free with semantic chunking methods like double-pass (uses spaCy to compute embeddings, and using a different embedding calculation method may increase costs) and classical sentence-based chunking. Methods utilizing embeddings (token-based and semantic percentile-based chunking) incurred costs lower than 0.01 USD. However, significant costs arose with the proposition-based chunking method: the approach using gpt-3.5-turbo costed 0.29 USD. This is nothing compared to generating chunks using gpt-4-turbo and gpt-4, which incurred costs of 17.80 and 29.33 USD, respectively.
Chunking type | Mean correctness score | Mean relevancy score | Mean faithfulness score | Mean semantic similarity score |
Token-based | 3,477 | 0,841 | 0,977 | 0,894 |
Sentence-based | 3,522 | 0,932 | 0,955 | 0,893 |
Semantic percentile | 3,420 | 0,818 | 0,955 | 0,892 |
Semantic double-pass merging | 3,682 | 0,818 | 1,000 | 0,905 |
Propositions-based gpt-3.5-turbo | 2,557 | 0,409 | 0,432 | 0,839 |
Propositions-based gpt-4-turbo | 3.125 | 0.523 | 0.682 | 0.869 |
Propositions-based gpt-4 | 3,034 | 0,568 | 0,887 | 0,885 |
We can see that the semantic double-pass merging chunking algorithm achieves the best results for most metrics. Particularly significant is its advantage over classical semantic chunking (semantic percentile) as it represents an enhancement of this algorithm. The most important statistic is the mean correctness score, and it is on this metric that the superiority of the new approach is evident.
Surprisingly, the proposition-based chunking methods achieved worse results than the other methods. RAG based on chunks generated with the help of gpt-3.5-turbo turned out to be very weak in the context of the analyzed text, as seen in the above table. However, RAGs based on chunks created using gpt-4-turbo/gpt-4 proved to be more competitive, but still fell short compared to the other methods. It can be concluded that chunking methods based on propositions are not the best solution for long prose texts.
Applying different chunking methods to texts with diverse characteristics allows us to draw conclusions about each method’s effectiveness. From the test involving chunking a straightforward text with distinct topic segments, it’s evident that clustering-based chunking is totally unsuitable as it loses sentence order. Classical chunking methods like sentence-based and token-based struggle to properly divide the text when segments on different topics vary in length. Classical semantic chunking performs better but still fails to perfectly chunk the text. Semantic double-pass merging chunking flawlessly handled the simple example.
Chunking a text containing pseudocode focused on comparing semantic chunking methods: percentile-based, double-pass, and proposition-based. Semantic chunking with a breakpoint set by percentiles couldn’t chunk the text optimally for any breakpoint value. Even for values allowing chunking of regular text (i.e., settings like in the first test), the method struggled, creating new chunks in the middle of pseudocode fragments. Semantic double-pass merging and propositions-based chunking using gpt-4 performed admirably, creating thematically coherent chunks.
A test conducted on a long prose text primarily focused on comparing metrics offered by LlamaIndex, revealing statistical differences between methods. Semantic double-pass merging and proposition-based method using gpt-4 generated the longest chunks. The fastest were classical token-based and sentence-based chunking due to their low computational requirements. Next were the two semantic chunking algorithms: percentile-based and double-pass chunking, which took twice as long. Proposition-based chunking took significantly longer, especially when using gpt-4 and gpt-4-turbo. This method, using these models, also incurred significant costs.
The free tested chunking methods were sentence-based and semantic double-pass merging chunking. Nearly cost-free methods were those based on token counting: token-based chunking and semantic percentile-based chunking. Comparing statistical metrics for RAGs created based on chunks generated by the aforementioned methods, semantic double-pass merging chunking performs best in most statistics. It’s notable that double-pass outperformed regular semantic percentile-based chunking as it’s its enhanced version. Classical chunking methods performed averagely, but far-reaching conclusions cannot be drawn about them because the optimal chunk length may vary for each text, drastically altering metric values. Proposition-based chunking is entirely unsuitable for chunking longer prose texts. It statistically performed the worst, taking significantly longer and being considerably more expensive.
Learn how to pick best textual data chunking method to lower processing costs and maximize efficiency!
Read more
In today’s digital landscape, the management and analysis of textual data have become integral to numerous fields, particularly in the context of training language models like Large Language Models (LLMs) for various applications. Chunking, a fundamental technique in text processing, involves splitting text into smaller, meaningful segments for easier analysis.
While traditional methods based on token and sentence counts provide initial segmentation, semantic chunking offers a more nuanced approach by considering the underlying meaning and context of the text. This article explores the diverse methodologies of chunking and aims to guide readers in selecting the most suitable chunking method based on the characteristics of the text being analyzed. The importance of this is particularly evident when utilizing LLMs to create RAGs (Retrieval-Augmented Generative models). Additionally, it dives into the intricacies of semantic chunking, highlighting its significance in segmenting text without relying on LLMs, thereby offering valuable insights into optimizing text analysis endeavors.
Chunking, in its essence, involves breaking down a continuous stream of text into smaller, coherent units. These units, or „chunks,” serve as building blocks for subsequent analysis, facilitating tasks such as information retrieval, sentiment analysis, and machine translation. The effectiveness of chunking is particularly important in crafting RAG (Retrieval-Augmented Generation) models, where the quality and relevance of the input data significantly impact model performance. This happens because different embedding models have different maximum input lengths. While conventional chunking methods rely on simple criteria like token or sentence counts, semantic chunking takes a deeper dive into the underlying meaning of the text, aiming to extract semantically meaningful segments that capture the essence of the content.
Before diving into the main body of the article, it’s worth getting to know a few definitions/concepts.
Text embeddings are numerical representations of texts in a high-dimensional space, where texts with similar meanings are closer to each other. In this space, each dimension corresponds to a word or token from the vocabulary. These representations capture semantic relationships between texts, allowing algorithms to understand language semantics.
Figure 1 Word embeddings - Source.
Cosine similarity is a measure frequently employed to assess the semantic similarity between two embeddings. It operates by computing the cosine of the angle between two vector embeddings that represent these sentences in a high-dimensional space. These vectors can be represented in 2 ways:
You can find more information about the differences between sparse and dense vectors here. This similarity measure evaluates the alignment or similarity in direction between the vectors, effectively indicating how closely related the semantic meanings of the sentences are. A cosine similarity value of 1 suggests perfect similarity, implying that the semantic meanings of the sentences are identical, while a value of 0 indicates no similarity between the sentences, signifying completely dissimilar semantic meanings. Additionally, an exemplary calculation along with an explanation is well presented in following video.
Figure 2 Sample visualization of cosine similarity - Source.
In Language Modeling (LLM), a context window refers to a fixed-size window that is used to capture the surrounding context of a given word or token in a sequence of text. This context window defines the scope within which the model analyzes the text to predict the next word or token in the sequence. By considering the words or tokens within the context window, the model captures the contextual information necessary for making accurate predictions about the next element in the sequence. It’s important to note that various chunking methods may behave differently depending on the size and nature of the context window used. The size of the context window is a hyperparameter that can be adjusted based on the specific requirements of the language model and the nature of the text data being analyzed. For more information about context window check this article.
Figure 3 Sample visualization of context window - Source.
Among chunking methods, two main subgroups can be identified. The first group consists of conventional chunking methods, which split the document into chunks without considering the meaning of the text itself. The second group consists of semantic chunking methods, which divide the text into chunks through semantic analysis of the text. The diagram below illustrates how to distinguish between various methods
Figure 4. Diagram representing the difference between the selected types of chunking.
Source-text-based chunking involves dividing a text into smaller segments directly based on its original form, disregarding any prior tokenization. Unlike token-based chunking, which relies on pre-existing tokens, source-text-based chunking segments the text purely based on its raw content. This method allows for segmentation without consideration of word boundaries or punctuation marks, providing a more flexible approach to text analysis. Additionally, source-text-based chunking can employ a sliding window technique.
This involves moving a fixed-size window across the original text, segmenting it into chunks based on the content within the window at each position. The sliding window approach facilitates sequential segmentation of the text, capturing local contextual information without relying on predefined token boundaries. It aims to capture meaningful units of text directly from the original source, which may not necessarily align with token boundaries. However, a drawback is that language models typically operate on tokenized input, so text divided without tokenization may not be an optimal solution.
It’s worth mentioning that LangChain has a class named CharacterTextSplitter
, which might suggest splitting text character by character. However, this is not the case, as this function splits the text based on the regex provided by the user (e.g., by space or newline characters). This is because each splitter in LangChain inherits from the text_splitter class, which takes chunk_size
and overlap as arguments. Subclasses override the split_text
method in a way that may not utilize the parameters contained in the base class.
Token-based chunking is a text processing method where a continuous stream of text is divided into smaller segments using predetermined criteria based on tokens. Tokens, representing individual units of meaning like words or punctuation marks, play a crucial role in this process. In token-based chunking, text segmentation occurs based on a set number of tokens per chunk. An important consideration in this process is overlapping, where tokens may be shared between adjacent chunks.
However, when chunks are relatively short, significant overlap can occur, leading to a higher percentage of repeated information. This can result in increased indexing and processing costs for such chunks. While token-based chunking is straightforward and easy to implement, it may overlook semantic nuances due to its focus on token counts rather than the deeper semantic structure of the text. Nonetheless, managing overlap is essential to balance the trade-off between segment coherence and processing efficiency. This function is built into popular libraries LlamaIndex and LangChain.
Sentence-based chunking is a fundamental approach in text processing that involves segmenting text into meaningful units based on sentence boundaries. In this method, the text is divided into chunks, with each chunk encompassing one or more complete sentences. This approach leverages the natural structure of language, as sentences are typically coherent units of thought or expression. Sentence-based chunking offers several advantages, including facilitating easier comprehension and analysis by ensuring that each chunk encapsulates a self-contained idea or concept. Moreover, this method provides a standardized and intuitive way to segment text, making it accessible and straightforward to implement across various text analysis tasks.
However, sentence-based chunking may encounter challenges with complex or compound sentences, where the boundaries between sentences are less distinct. In such cases, the resulting chunks may vary in length and coherence, potentially impacting the accuracy and effectiveness of subsequent analysis. Despite these limitations, sentence-based chunking remains a valuable technique in text processing, particularly for tasks requiring a clear and structured segmentation of textual data. Sample implementation is available in nltk.tokenize.
Recursive chunking is a text segmentation technique that employs either token-based or source-text-based chunking to recursively divide a text into smaller units. In this method, larger chunks are initially segmented using token-based or source-text-based chunking techniques. Then, each of these larger chunks is further subdivided into smaller segments using the same chunking approach. This recursive process continues until the desired level of granularity is achieved or until certain criteria are met. Its drawback is computational inefficiency.
Hierarchical chunking is an advanced text segmentation technique that considers the complex structure and hierarchy within the text. Unlike traditional segmentation methods that divide the text into simple fragments, hierarchical chunking examines relationships between different parts of the text. The text is divided into segments that reflect various levels of hierarchy, such as sections, subsections, paragraphs, sentences, etc. This segmentation method allows for a more detailed analysis and understanding of the text structure, which is particularly useful for documents with complex structures such as scientific articles, business reports, or web pages.
Hierarchical chunking enables the organization and extraction of key information from the text in a logical and structured manner, facilitating further text analysis and processing. An advantage of hierarchical chunking is its ability to effectively group text segments, particularly in well-formatted documents, enhancing readability and comprehension. However, a drawback is its susceptibility to malfunction when dealing with poorly formatted documents, as it relies heavily on the correct hierarchical structure of the text. LangChain comes with many built-in methods for hierarchical chunking, such as MarkdownHeaderTextSplitter, LatexTextSplitter, and HTMLHeaderTextSplitter.
Semantic chunking is an advanced text processing technique aimed at dividing text into semantically coherent segments, taking into account the meaning and context of words. Unlike traditional methods that rely on simple criteria such as token or sentence counts, semantic chunking utilizes more sophisticated techniques of semantic analysis to extract text segments that best reflect the content’s meaning. To perform semantic chunking, various techniques can be employed. As a result, semantic chunking can identify text segments that are semantically similar to each other, even if they do not appear in the same sentence or are not directly connected.
Semantic chunking using k-means involves a multi-step process. Firstly, sentence embeddings need to be generated using an embedding model, such as Word2Vec, GloVe, or BERT. These embeddings represent the semantic meaning of each sentence in a high-dimensional vector space. Next, the k-means clustering algorithm is applied to these embeddings to group similar sentences into clusters. Implementing semantic chunking with k-means requires a pre-existing embedding model and expertise in NLP and machine learning. Additionally, selecting the optimal number of clusters (k) is challenging and may necessitate experimentation or domain knowledge.
One significant drawback of this approach is the potential loss of sentence order within each cluster. K-means clustering operates based on the similarity of sentence embeddings, disregarding the original sequence of sentences. Consequently, the resulting clusters may not preserve the chronological or contextual relationships between sentences. We strongly advise against using this method for text chunking when constructing RAGs. It leads to the loss of meaning in the processed text and may result in the retriever returning inaccurate content.
This chunking strategy explores leveraging LLMs to discern the optimal content and size of text chunks based on contextual understanding. At the beginning, the process involves creating so-called “propositions”, often facilitated by tools like LangChain. Propositions are defined as atomic expressions within text, each encapsulating a distinct factoid and presented in a concise, self-contained natural language format.
These propositions are then passed to an LLM, which determines the optimal grouping of propositions based on their semantic coherence. Performance of this approach heavily depends on the language model the user chooses. Despite its effectiveness, a drawback of this approach is the high computational costs incurred due to the utilization of LLMs. Extensive explanation of this method is in this article and a modified proposal is presented in this tweet.
This semantic chunking implementation utilizes embedding models to determine when to segment sentences based on differences in embeddings between them. It operates by identifying differences in embeddings between sentences, and when these differences exceed a predefined threshold, the sentences are split. Segmentation can be achieved using percentile, standard deviation, and interquartile methods. A drawback of this approach is its computational complexity and the requirement for an embedding model. This algorithm’s implementation is available in LlamaIndex. Greg Kamradt showcased this idea in one of his tweets.
Considering the challenges faced by semantic chunking using various mathematical measures (standard deviation/percentile/interquartile), we propose a new approach to semantic chunking. Our approach is based on cosine similarity, and the initial pass operates very similarly to the previously described method. What sets it apart is the application of a second pass aimed at merging chunks created in the first pass into larger ones. Additionally, our method allows for looking beyond just the nearest neighbor chunk.
This is important when the text, which may be on a similar topic, is interrupted with a quote (which semantically may differ from the surrounding text) or a mathematical formula. The second pass examines two consecutive chunks: if no similarity is observed between the two neighbors, it checks the similarity between the first and third chunks being examined. If these two chunks are classified as similar, then all three chunks are merged into one. A detailed description of the algorithm and code will be presented in an upcoming article, which will be published shortly.
As you can see there are many diverse chunking algorithms differing in various aspects such as required computational power, costs, duration, and implementation complexity. Selection of an appropriate chunking algorithm is an important decision as it impacts two key factors of the solution: quality of final results (quality of answers generated by RAG) and cost of running it. Therefore, it should be preceded by a thorough analysis of, amongst others, the purpose for which the chunking is to be performed and quality of source documents. Our next article comparing the performance of various chunking methods can be helpful with taking a decision. Stay tuned!
Explore available chunking methods and how they work!
Read more
In the world of data science and technology, one cannot ignore the allure of Large Language Models (LLMs). Their capabilities are undeniably captivating for enthusiasts in the field. However, despite the excitement, caution should be exercised. Let’s talk about when it’s not advisable to use LLMs in your data science projects.
As we all know, Large Language Models are trained on a massive amount of data so that they can perform a variety of tasks, allowing users to save a significant amount of time. They provide higher-quality outputs in tasks like translation, text generation, and question answering, compared to, for example, rule-based systems where developers manually create rules and patterns for language understanding. Conversely, if your data science project involves highly technical or specialized content, using a pre-trained LLM alone may result in inaccurate or incomplete results. In such cases, incorporating domain-specific models or knowledge bases may be necessary. To accomplish this, a substantial amount of data is essential, given that these models possess billions of parameters. Effective fine-tuning requires a significant quantity of data.
Consequently, if there is an awareness that the data available is limited, or if there are constraints on the data, it is advisable to first consider an approach utilizing Natural Language Processing (NLP). In such cases, an NLP model or less complex LLM, which is also known as Small Language Model can still yield satisfactory results on the available dataset. Review our article about the advantages of using SLMs over LLMs: When bigger isn’t always better – Bring your attention to Small Language Models.
When discussing the drawbacks of Large Language Models, it is essential to mention one of the most common issues, namely the tendency of models to hallucinate. Anyone who has used or is using ChatGPT3.5 has undoubtedly experienced this phenomenon – simply put, it is the moment when the model’s responses are completely incorrect, containing untrue information, despite appearing coherent and logical at first glance. This is primarily influenced by the dataset on which the model was trained, as it is vast, originating from many sources that often may contain subjective, biased views, or distorted information.
The cause of hallucinations also lies in using models for tasks they were not adapted for. The feature which seems to be an advantage when it comes to creative tasks, such as composing songs and writing poems, becomes a disadvantage when we expect the model to provide only factual information. As we know, LLMs perform very well in general natural language processing tasks, so applying them to specific Data Science tasks will result in outcomes deviating from the truth. In such situations, it is necessary to tailor these models to a specific problem, armed with an adequate amount of high-quality data. As we know from the previous paragraph, acquiring such data is a challenging and laborious process. However, even if we manage to create such a dataset, the issue of fine-tuning the model still remains, posing an additional challenge if computational power and cost resources are limited.
LLMs also encounter challenges in processing streaming data. As we know, they are trained on texts of finite length (a few thousand tokens), resulting in a decrease in performance when handling sequences longer than those on which they were trained. The architecture of LLMs caches key-value states of all previous tokens during inference, consuming a significant amount of memory. As a result of this limitation, large language models face difficulties in handling systems that require extended conversations, such as chatbots or interactive systems.
It is worth noting that the StreamingLLM framework comes to the rescue in this context, where the authors leverage the initial tokens of LLMs to serve as the focal point for the allocation of attention scores by caching initial tokens alongside recent ones. Nevertheless, keep in mind that this framework does not extend LLMs context window – retaining only the latest tokens and attention sinks while discarding the middle ones.
Deploying LLMs in data science projects may raise legal and ethical challenges, especially when dealing with sensitive or regulated domains. LLMs can be vulnerable to attacks, where malicious actors intentionally input data to deceive the model. It is crucial to remember that the model’s responses may contain inappropriate or sensitive information.
The absence of proper data filtering or management can lead to the leakage of private data, exposing us to the risk of privacy and security breaches. The recent inadvertent disclosure of confidential information by Samsung employees highlights significant security concerns associated with the use of Large Language Models (LLMs) like ChatGPT. Samsung’s employees accidentally leaked top-secret data while seeking assistance from ChatGPT for work-related tasks.
The incident serves as a stark reminder that any information shared with these models is retained and utilized for further training, raising privacy and data security issues. This incident not only demonstrates the unintentional vulnerabilities associated with using LLMs in corporate settings but also underscores the need for organizations to establish strict protocols to safeguard sensitive data. It emphasizes the delicate balance between leveraging advanced language models for productivity and ensuring robust security measures to prevent inadvertent data leaks.
Another important aspect is that LLMs generate responses that are non-interpretable and unexplainable. Large Language Models are often referred to as black boxes, as it is often impossible for users or even the creators of the model to determine exactly what factors influenced a particular response. Additionally, there may be cases where the same question yields different answers, which is unacceptable for certain use cases.
Therefore, if project requirements include a transparent and logical decision-making process, relying on responses from a language model is not advisable. However, it is still worth considering eXplainable Artificial Intelligence (XAI) in Natural Language Processing (NLP) for such problems. Explore the role of XAI in addressing the interpretability posed by machine learning models in another of our insightful articles: Unveiling the Black Box: An overview of Explainable AI.
In situations where project requirements involve processing responses in real-time, large language models are not a suitable choice. They possess an enormous number of parameters, translating into a significant demand for computational power for processing. The computational load of large models can be prohibitive. Due to the high complexity, large language models often exhibit extended inference times, introducing delays that are unacceptable in real-time contexts. Applications processing vast amounts of data in real-time, given their flexibility and the tendency for context changes in text, would require continuous fine-tuning to meet demands. This, in turn, results in substantial costs for maintaining model quality.
In summary, while large language models exhibit impressive language understanding, their practical implementation comes with challenges related to computational efficiency, latency, resource usage, scalability, unpredictability, interpretability, adaptability to dynamic environments, and the risk of biases. These factors should be carefully considered when deciding whether to use large language models in data science projects.
Large Language Models (LLMs) have amazing language comprehension, but their practical usage can cause challenges related to efficiency, latency, resource usage, scalability and more!
Read more
Undoubtedly, there is a lot of hype around Large Language Models. We are pleased to observe what is happening and simultaneously gather knowledge and experience in the field. These powerful models have demonstrated their immense capabilities in a wide range of use cases, so our customers are also curious about new possibilities and eager to use in the projects popular large-scale models like ChatGPT. To the surprise of our clients, it is not always the best choice.
In a world where bigger is often perceived as better, perhaps it’s time to challenge this preconception – at least when it comes to Large Language Models. In this article, we’ll delve into scenarios in which opting for a more modestly sized LLM might prove to be the wiser and more pragmatic approach.
Large language models (LLMs) are characterized by a significant increase in the number of parameters they possess, often reaching billions or even trillions. As the parameter count grows, these models tend to deliver greater accuracy and generate higher-quality outputs in tasks like translation, text generation, and question answering. Imagine GPT-3.5, developed by OpenAI, a powerful language model with 175 billion parameters. As the GPT series is expanding the GPT-4 is said to be based on eight models with 220 billion parameters each, which gives a total of about 1.76 trillion parameters, making it nearly 1000 times larger than the GPT-3.5. However, it is important to note that as LLMs grow, they bring along a set of challenges that must be acknowledged and considered.
The first challenge could be the cost, which depends on many factors. Primarily, LLMs can be distinguished for commercials and open source. In the case of commercial ones usually the cost is evaluated for each model usage based on the number of tokens used in its call. Even if the unit cost of the model usage is relatively small, for example gpt-3.5-turbo around $0.002 per 1000 tokens, the cost grows rapidly if you want to use the model a million times a day.
On the other hand, open-source models have no direct cost per request, they are generally free to use. Open-source LLMs expenses are related to the infrastructure. Simplifying, GPU memory requirements depend linearly on the number of model parameters. It can be assumed that storing a 1B parameter in GPU memory, required for inference — costs 4 GB at 32-bit float precision. Please find below the cost of some open-source models which can be run on the NC A100 v4 series.
Model name | Size | Cluster | GPU | Cost |
LLaMA2–7B | 7b parameter | NC24ads A100 v4 | 1X A100 | $3.67/hour |
Dolly-v2-12b | 12b parameter | NC24ads A100 v4 | 1X A100 | $3.67/hour |
LLaMA-2–70b | 70b parameter | NC48ads A100 v4 | 2X A100 | $7.35/hour |
Smaller LLMs offer a more efficient alternative, allowing for computing and training on less powerful hardware. Sometimes it is possible to self-host such a model on a private machine instead of using computational server, but we need to be sure to provide minimum system requirements to do so. In the end, the number of requests or the usage volume is a critical factor in determining the real cost for a given use case.
When we think about resources, environmental aspects are also an advantage, as using smaller models creates a smaller carbon footprint.
Despite the fact that pre-trained LLMs can provide valuable insights and generate text in various domains, they may lack the domain-specific knowledge required for certain specialized tasks. In the realm of data science projects, where the focus is on addressing specific business needs, the relevance of information concerning distinctions between butter and margarine, or the causes of the French Revolution, is not evident. While information from diverse set of areas such as cuisine or history can be insightful, they may not be pertinent to business clients seeking solutions tailored to their specific tasks. Not every project requires the vast knowledge and generative abilities of billion-parameter LLMs.
If your data science project involves highly technical or specialized content, using a pre-trained LLM alone may result in inaccurate or incomplete results. In such cases, incorporating domain-specific models or knowledge bases may be necessary. Smaller models can be tailored to specific use cases more effectively. They allow data scientists to fine-tune the model for particular tasks, resulting in better performance and efficiency.
Massive models can introduce delays in processing due to their size and complexity. Generally, smaller language models provide responses faster than larger models. This is because smaller models have fewer parameters and require less computational power to generate responses. They can process and generate text more quickly, making them a preferred choice for applications where low latency is important. Let’s see the difference in OpenAI models we mentioned earlier. One of the experiments comparing response time for these models result in following:
The trade-off between response speed and response quality needs to be carefully considered when choosing a model for a specific application. The choice of model size should align with the specific requirements and constraints of the project.
With all that said, we hoped to expand your perspective on the language models and the idea that larger models may not always be a better one. When considering an LLM for your data science project, it’s essential to evaluate the specific requirements of your task and weigh them against the potential drawbacks of using a massive model. Smaller LLMs offer practical advantages in terms of computational efficiency, cost-effectiveness, environmental sustainability, and tailored performance, despite their own disadvantages and limitations.
In 2023 LLMs became symbol of AI capability. But they are not always the best solution for your AI needs. Why? Read the article and find out!
Read more
At BitPeak, we are happy to see the growing importance and strategic approach to data, analytics & cloud landscape among financial & insurance companies. The 2023 FinTech & InsurTech Digital Congress emerged as an important forum on the future of the industry. It was an opportunity to discuss key trends and their impact on the sector, with an emphasis on technology & AI. Topics ranged from operational challenges in adapting to industry transformations to innovative strategies for navigating the evolving technological landscape, capped by a vision for the future of FinTech & InsurTech. In this article you can read the brief summary of the topics discussed on the summit to keep on the top of rapidly changing landscape of technological solutions and visions in the finance sector.
The Congress started with a focus on the future. The introductory speech was held by the Congress’ Board Chairs Marcin Petrykowski and Jan Kastory. They highlighted four key market trends: Constant Evolution vs Revolution, AI’s Role, Financial Markets being challenged, and the Rise of Embedded & Decentralized Finance. Looking forward to 2024, the focus of the sector revolves around “Revive, Grow, and Prepare”, with each company facing a decision where to put the emphasis in its strategy. Next edition of FinTech & InsurTech Digital Congress will be a good opportunity to look back and verify these forecasts.
Agnieszka Jadczyszyn, the Operations Head at mBank, highlighted the challenges from bank’s operations department perspective. As the financial world changes, she shared insights into adapting and building resilience during times of market transformation.
Marek Myszka, Head of Innovation at PKO Bank Polski, focused on leveraging gaming platforms like Roblox and Fortnite in the Metaverse. Breaking away from conventional trends, he highlighted the potential to capture a younger audience and refreshing brand identity, all while navigating the complexities and opportunities presented by the Metaverse. Marek Myszka and his team seize the opportunity on smaller Metaverse hype to gain expertise and build new use cases following a steady pace – interesting case how a potential risk can be turned into opportunity.
Paulina Skrzypińska, Chief Innovation Officer at BNP Paribas Bank Polska, shared insights into the importance of tech education within teams and harnessing existing resources. Drawing attention to practical challenges in implementing AI use cases and the need for industry-wide synergy, she emphasized how collaborative innovation, rather than isolation and silos, is the key to navigating the evolving technological landscape – with BLIK being a textbook example of how the sector should cooperate to bring innovation.
The panel on Customer Experience brought to the forefront the evolving dynamics of customer interactions. From intertwining experiences in various services to the increasing importance of Employee Experience, the session explored the post-COVID imperative of empathy in customer relations. The discussion culminated in the potential industry shift towards a comprehensive 360-degree insurance offering tailored for risk-averse customers.
In the session on the future landscape of financial institutions’ data access in 2030, Dr. Krzysztof Korus highlighted the key legislative changes. The discussion touched upon regulations such as PSD2, FIDAR, Data Act, DGA, and GDPR. Dr. Korus highlighted the differences between Open Banking and Open Finance, emphasizing that while Open Banking operates through free APIs for both reading and writing, Open Finance follows a structured, paid model with read-only access. Notably, the EU regulation requires the creation of a nationwide OpenFinance platform within the next 18 months. The goal is to facilitate secure and standardized data exchange, marking another step in the evolution in financial data accessibility.
As we wrap up our coverage of the 2023 FinTech & InsurTech Digital Congress, we’re left with a clearer view of where the financial and insurance sectors are heading. This congress has showcased a range of perspectives, from the use of gaming platforms in banking to the evolving nature of customer service and the implications of upcoming data regulations. These discussions provide a roadmap for navigating the future challenges and opportunities in FinTech and InsurTech. Looking ahead, it’s evident that these industries are on a path of continuous innovation, adapting to meet the demands of a rapidly changing technological landscape. But fortunately for those wanting to be up to date, #BitHikers will provide insights and summaries of the most important trends and events in the data tech industry.
BitPeak at the 2023 FinTech and InsurTech Congress - an amazing opportunity to see and discuss the newest trends in the impact of technology on the sector.
Read more
The IIBA Poland 2023 Summit gathered approximately 200 people associated with Business Analysis in Poland. The conference provided a unique opportunity to learn about the latest trends, insights, and strategies within the field of Business Analysis. One of the main topics of the event was integration of Artificial Intelligence (AI) into the toolkit of business analysts. Speakers shed some light on the associated benefits and challenges. Additionally, a spectrum of solutions employed by business analysts was showcased during the presentations.
Presented by Susan Moore, the speech titled “Navigating the Intersection of AI and Business Analysis Work” provided a glimpse into the creative potential of AI. Ms. Susan demonstrated how AI could not only generate professional text information, such as case stories and defined business rules for specific scenarios but also poems that mimic the style of famous writer.
The integration of artificial intelligence into the field of Business Analysis has the potential to change processes of information gathering, scenario creation and requirements analysis. It can serve as a powerful tool for speeding up tasks, testing ideas, and revealing the pros and cons of various approaches. However, the promises of AI come with a fair share of responsibilities. The presenters in the AI block emphasized the importance of carefully verifying information obtained from AI systems. They correctly pointed out that AI-generated responses, while generally reliable, can sometimes lead to misinterpretation or misdirection when looking for specific details.
The next significant concern discussed was the challenge of bias. AI’s knowledge is derived from the data it has been trained on, and it tends to use a statistical approach, often leading to the production of stereotypically biased responses. For example when asking for a photo of a typical Business Owner, it is likely that AI systems based on pre-existing biases will then generate images of an older white man. To address this pressing challenge, diverse datasets and vigilant human supervision are necessary, ensuring the ethical use of AI. This highlights the responsibility that accompanies AI adoption, as machines are devoid of emotions or ethical judgment.
Additionally, AI, however advanced, has its boundaries. It cannot digitize every form of human knowledge, especially the intricate aspects of human inspiration and behavior. AI can only mimic people in the range limited by possessed information, for example, creating poems in the style of a writer it knows. In these situations, experienced business analysts can assist in understanding complex human problems and formulate relevant questions, especially when essential information is not available in well-structured formats. This highlights the irreplaceable role of human cognition in certain domains of business analysis.
Another speaker, Marcin Żmigrodzki, delved into the practical applications of AI within business analysis, focusing on “AI Support for Business Tasks”. He rightfully highlighted that Artificial Intelligence excels in typical and standard systems but faces challenges with unique and customized systems with complex entity relationships and dependencies. AI systems like Chat GPT are adept at providing answers to general questions but might hallucinate when it comes to specific and detailed inquiries.
Artificial Intelligence capabilities shine in tasks like filtering large datasets for relevant features and quickly summarizing extensive texts in various formats, such as legal documents, images, and unstructured data, providing structured and synthesized information. Nevertheless, AI-generated responses are often based on shallow analysis and may struggle with revising information and comprehending complex schema texts due to their inherent limitations in symbolic thinking.
In conclusion, our exploration of AI’s role in business analysis indicates that we can use its power right now. However, we must be cognizant of its limitations. Nonetheless AI can serve as a valuable source of information, helping us in our tasks, and providing a fresh perspective on the issues we analyze. It can assist in debugging and offer broader insights into the problems we face, making it a powerful ally in our journey to enhance business analysis.
During the conference, the presentation by Anna Kochanowska turned out to be particularly intriguing. Her session revolved around the notion that “Product thinking is BA’s superpower”. In her own words, “Product Thinking” is the art of “doing now what the client needs next,” a concept that challenges our approach to work.
She delved into a topic that is often the bane of innovation – what she termed the “build trap”. This is the tendency of the project team to transform into a feature factory, focusing on delivering what customers explicitly request, all with a sense of routine. Such an approach stifles creativity, limits openness to new ideas and makes it difficult to meet the changing needs of customers.
“Product Thinking”, in contrast, appears to be a visionary solution, referring to the new paradigm of agility. At its core, it places customers at the center of attention. The product becomes a flexible entity, carefully tailored to the individual needs of the customer. The speaker emphasized that “Product Thinking” goes beyond the world of software and technology, recognizing that every client has a distinct perspective and preferences regarding the desired product. Moreover, every component influencing product consumption and value delivered is carefully considered and integrated. This holistic mindset leads to better user experiences and products that meet a variety of needs of customers.
The essence of “Product Thinking” lies in its commitment to challenge every aspect of the final product, from requests, through ideas to requirements. This requires a continuous process of exploration and validation, manifested by solution testing and experimentation. “Product Thinking” emphasizes an open-mindedness, recognizing that answers can be obtained from unconventional sources, often from people outside the immediate team or enterprise. This cultivation of open thought processes fosters increased creativity and novelty, resulting in solutions that deliver significant business value.
Anna Kochanowska emphasized that within the realm of “Product Thinking”, the principle is to break free from routine and adopt a daring and experimental mindset. This approach imitates the role of a crazy scientist, who is not afraid to explore uncharted territories and discover unconventional ideas. It is an environment where risk-taking and creative thinking are valued. The presentation highlighted the significance of each team member’s contribution, emphasizing that facilitating collaboration is an important pillar of “Product Thinking”. This holistic and customer-centric approach empowers Business Analysts to proactively meet client needs, sometimes even exceed expectations, and become drivers of positive change within their organizations.
The IIBA Poland Summit 2023 served as an informative journey into the constantly developing sphere of Business Analysis. For professionals in this field, it offered a valuable repository of insights and discussions that will undoubtedly shape the future of this discipline.
During the conference, participants engaged in substantive dialogues, delving into the subjects that took center stage, including the integration of AI and the role of Product Thinking. These discussions not only contributed to a deeper understanding of these key topics, but also enabled the exchange of views and finding common points among attendees. Outside the lecture hall
However, despite many positives, there was a small flaw in the image of the conference. Namely, parallel sessions and the lack of previously available abstracts of the presentations did not allow me to properly plan my participation in the conference. This limitation impacted the ability to choose the speeches with the highest business value.
As a #BitHiker, combining technological solutions with a business perspective is a fundamental principle of our identity. Using our extensive technical knowledge and expertise, we are well prepared to face the challenges related to the sphere of Business Analysis.
The most important points from recent premier Business Analytics conference in Poland - IIBA Summit 2023
Read more
Are you considering the implementation of a business intelligence tool but find it challenging to select the right one? There are multiple options available on the market, so the choice might be difficult, as not every piece of information is easily accessible or clear. Additionally small details can have a future impact on scalability, costs or ability to integrate other solutions. But you are in luck as our experts are ready to provide you with guidance and a comparison of three distinct BI systems, to help you make a more informed choice.
Power BI, created by Microsoft, is a very user-friendly business intelligence tool. It enables you to easily import data from various sources and create interactive dashboards as well as reports. Its drag-and-drop interface makes it accessible to non-technical users and allows it to work well in self-service scenarios. Additionally, this tool is also very robust when it comes to enterprise-grade solutions.
Being a part of Microsoft’s ecosystem is one of its strongest points as it seamlessly integrates with the whole suite of Microsoft products like Excel, Power Point, Teams and Azure. It is also a key component in a brand-new data platform called Microsoft Fabric!
Tableau is one of the first players when it comes to BI tooling on the market. It empowers users to explore and understand data through interactive and shareable dashboards. Tableau also supports data integration from multiple sources, offering visually appealing and complex visualizations. The ability to create very sophisticated visualizations which can reveal hidden business insights is Tableau’s most recognizable trademark.
Additionally, this tool encourages collaboration, making it suitable for teams to share insights and work on data projects. Currently owned by Salesforce, it easily integrates with this most popular CRM system on multiple levels.
Wyn Enterprise might be the least known of the three, but it has some unique approach amongst BI tooling. Let’s start by saying that it is a comprehensive business intelligence and reporting platform designed for enterprise-level data analysis and provides robust data integration capabilities, customizable reporting, and dashboarding options.
It prioritizes security and governance, making it suitable for large organizations with strict data compliance requirements. The main focus of this solution are embedding scenarios for a vast number of users. Combine it with exceptionally attractive licensing and you have a very good combo for many organizations!
Let’s explore how the tools stack up when it comes to data preparation, connectivity, automation and scalability.
Having out-of-the-box data connectors and the ability to shape the data is crucial for smooth and effective workflow. This is especially important when working with excel or csv files. But even with database as a source, small tweaks in data are often necessary. A tool that allows the user to quickly connect to particular data sources and transform data to correct format without the need to use other tools is a blessing, increasing the efficiency and easy of use of the whole system.
Well-prepared data is the basis for proper analysis and thus for correct business information. Properly modeled and mapped data can contribute to the correct calculation of key business KPIs.
Looking at Power Bi, typically the first component users interact with is Power Query. And this is great because Power Query can be also found in Excel ( the most popular analytical tool on our planet btw.) and is well known among its users. Power Query is also praised both for its intuitive GUI and for its M language which offers great flexibility for data transformations.
On the other hand, Tableau has its own offering called Tableau Prep which is highly appreciated for its extensive use of AI in suggestions for data transformation processes. This helps the users to speed up work time and take advantage of facilities that he would not have noticed. In addition, most things can be done using a graphical interface, without any code. Wyn Enterprise provides some data preparation options, although in a more limited capacity. So preferably, it would be used with data that is already clean and transformed.
All three tools come equipped with a diverse array of data connectors, ensuring effortless integration with popular databases. They each support both scheduled and incremental refresh options, enabling users to keep their data current. Furthermore, they provide flexibility in selecting various connection types tailored to specific requirements.
A noteworthy feature shared by Tableau and Wyn Enterprise is the absence of any limits on data input size. This means your data can scale in tandem with your business growth, free from constraints. Additionally, all three tools are equipped with incremental refresh capabilities, resulting in efficient data updates and options to parametrize data sources, which greatly improves the experience of working with multiple data environments.
Data modeling is one of the key things when working with data. Starting with any work, architects, bi-developers, data engineers and data modelers face the challenge of creating a model that fully meets business requirements. This can be difficult, especially with large and complex models based on different data sources. In this case, we expect that the BI tool supports developer in this task and offers the highest possible data processing performance. So, we would like to compare Tableau, Power BI and Wyn Enterprise applications in the most important aspects for us from the developer’s point of view.
All of the aforementioned software offers the possibility of modeling and creating relationships between tables. They all work best together in the context of efficiency and optimization in the structure of star schema. All of the three tools allow you to create measures prepared for specific business requirements. Power BI and Wyn have very similar analytical languages, with the same concepts such as context and context transition. Although there are some differences in the number of functions available (in favor of Power BI). Tableau offers VizQl which is really similar to SQL language which we use in database. That makes it easier for people switching from a database to BI application.
The reporting layer is very important as it touches both report developers, who create complex dashboards based on gathered requirements, and business stakeholders who use those dashboards on a daily basis. Therefore, reporting capabilities must fulfill the needs of both groups. For developers the tool needs to be flexible, easy to use and with vast amounts of functionality.
Having those attributes results in a data product (report, dashboard) that will be used on a daily basis by the Business and will grant observability, deliver insights or just plainly make their life easier when it comes to running their company.
We can clearly say that in this category Tableau is ahead of the competition. It is following a grammar of graphics approach where visuals can be built layer by layer. Some things that are easily achieved in Tableau are out of reach when using Power Bi or Wyn Enterprise. Power BI is currently investing heavily in its native visuals and its reporting capabilities so we can clearly expect some great features in the coming months. It is also worth mentioning that Wyn Enterprise has more out-of-the-box visuals than Power BI at this moment.
We’ve prepared a detailed comparison of available features:
The ability to share reports, manage access and allow users to see only the relevant data is basically the main difference that distinguishes BI tools from non-BI ones, such as MS Excel. In the world of Excel, spreadsheets can be sent or shared without any restrictions. Typically, users can modify the data, perform their own detailed analysis and suddenly what happens is that we have multiple versions of the same file flying around and nobody knows which one is the right one. A true nightmare.
With BI systems like Power BI, Tableau or Wyn Enterprise it should not happen as those tools have built-in sharing functionalities, access management, security, data loss prevention and many more. Business users wouldn’t be able to modify the underlying data but will be able to perform their own analysis using available models. Perfect!
The second thing that is worth keeping an eye on is what happens with your data assets, as they are crucial to get the most out of your BI solutions. Let’s imagine a real-life situation. You worked hard to ingest all the relevant data, transformed it, modeled it by applying all the hard gathered business logic, created splendid dashboards and you think you can rest now?
Well, not really… Truth is that there might be a possibility that end-users are not using your data product as it doesn’t bring them any kind of business value. To know that it is the case and to react quickly by adjusting final solution you need to have some observability of what is going on. You would like to monitor usage rates and also get relevant feedback from end users.
AI! The new word of the year. If you are not sleeping under a rock, then you know we couldn’t omit it in our analysis. AI-based solutions are being added to almost every tool to increase development speed and/or increase user experience. AI features can be divided into the ones that use simpler ML algorithms and the ones based on modern Large Language Models.
The first group has been available in many BI tools for several years – mainly in the form of more sophisticated charts that could reveal some hidden insights or as interface where users could ask the question about data (with really mixed results). The second group is being introduced as we speak.
It brings the promise of huge productivity boost for both report developers and business users. Available previews show that LLMs could help developers with building report elements, generating code and performing deeper analysis. Business users would be able to ask questions about data, receive report summaries or insights-based recommendations.
The changes are both rapid and promising, so it is important to watch out for new tools and implementations. But for now, let’s focus on the comparison of existing features
Both Microsoft and Salesforce are heavily investing in this domain so in Power BI we will have Copilot serving both developers and users and in Tableau we will have Einstein Copilot (for developers) and Tableau Pulse (for business users).
As you can see, each solution has its strengths. The choice is not easy and should always take into consideration needs, means and perspectives of an organizations. But with our guide (that you can always go back to!) You should be able to decide on the path that will result in highest efficiency and scalability, as well as lowest costs!
Are you considering the implementation of a business intelligence tool but find it challenging to select the right one?? Read the article and be learn a Bit about possible tools, their characteristics and comparisons!
Read more
In this comprehensive guide, we delve into the critical realm of data quality assurance using dbt (data build tool). Data quality is paramount in the world of data analytics and decision-making. To ensure the reliability, accuracy, and consistency of your data models, you need a robust testing framework and a well-organized project structure.
Here are the key files and directories you’ll be working with in a dbt project:
By understanding the key files and directories in your dbt project, you can effectively organize, manage, and scale your data transformation processes while ensuring data quality in your project.
Dbt’s testing framework is designed to ensure data quality and consistency by validating the data within your models. It provides built-in tests, as well as the ability to create custom tests tailored to your specific data requirements. The testing framework is an essential component of any dbt project as it promotes trust in your data and helps identify issues early in the development process.
dbt’s testing framework includes the following components:
Generic Tests:
These are predefined tests that validate the structure of your data. Initially, there are four of them but you can create and add more. The initial four are:
You can configure generic tests in the schema.yml file which is associated with your models.
Custom Data Tests:
Custom data tests allow you to define your own SQL queries to test specific data requirements not covered by generic tests. These tests are written in individual SQL files and stored in the tests directory of your dbt project. When creating custom data tests, ensure the SQL query returns zero rows for a successful test or one or more rows for a failed test.
Test Configuration:
dbt allows for configuration of your tests by setting test severity levels, adjusting error thresholds, or even disabling specific tests. These configurations can be defined in the dbt_project.yml file or directly within the schema.yml file for individual tests.
Test Execution:
To execute tests in dbt, use the dbt test command. This command runs all the tests defined in your project, including schema, and custom data tests. The results are displayed in the console, indicating the success or failure of each test, along with any relevant error messages.
Test Documentation:
dbt 's testing framework also integrates with other feature. When generating documentation for your project, the test information is included in the generated documentation, providing a comprehensive overview of quality checks performed on your data models.
By integrating data tests into your development workflow, dbt’s testing framework empowers you to actively safeguard the reliability and accuracy of your data models. This proactive approach ensures that potential data issues are identified and rectified early in the development process, preventing inaccuracies and inconsistencies from proliferating through your data pipeline. As a result, you can trust that your data models consistently produce high-quality, dependable insights crucial for informed decision-making.
Setting up a testing environment for your dbt project is crucial to ensure data quality and integrity. Here are some tips to help you create an efficient and effective testing environment:
By following these tips, you can set up a robust testing environment that helps ensure the quality and integrity of your dbt project, allowing you to build and maintain reliable, accurate, and valuable data models.
The dbt community has created several packages that extend the built-in testing capabilities and help improve data quality in your projects. These packages offer additional tests, macros, and utilities to help you effectively manage your testing process. Some popular community-made testing packages include:
dbt-utils: The dbt-utils package is a collection of macros and tests which can be used across different projects. It includes tests for handling more complex scenarios, such as testing whether a combination of columns is unique across a table or asserting that a column has values in a specified range. You can find the package on GitHub here
dbt-expectations: Inspired by the Great Expectations Python library, this package provides a suite of additional data tests to expand the built-in test functionality of dbt. It covers a wide range of data quality checks, such as string length tests, date and timestamp validations, and aggregate checks. The package is available on GitHub here
dbt-date: The dbt-date package is a collection of date-related macros designed to simplify working with date and time data in dbt projects. It includes macros for generating date ranges and creating date dimensions. It’s a very useful and readable abstraction that can help you create new tests relating to datetime fields in your models, as well as create the models themselves. You can find the package on GitHub here
dq-tools: The dq-tools package purpose it to provide an easy way for storing test results and visualizing them in a BI dashboard. The dashboard focuses on the six KPI’s mentioned in the previous article: accuracy, consistency, completeness, timeliness, validity, uniqueness. This package can be found on GitHub here
dbt-meta-testing: The dbt-meta-testing package is a tool for meta-testing your dbt project. It asserts test and documentation coverage. You can find the package on GitHub here
dbt-checkpoint: To use these packages in your dbt project, you need to add them as dependencies in your packages.yml file and run dbt deps to download and install them. Once installed, you can use the additional tests, macros, and utilities provided by these packages in your projects. You can find it on GitHub here
By leveraging community-made testing packages, you can enhance the testing capabilities of your dbt project, ensuring data quality and consistency throughout your data transformation processes.
Dbt’s testing framework ensures data quality and consistency by providing built-in tests, custom tests, test configuration, test execution, and test documentation. Implementing data tests in the development process ensures data models remain reliable and accurate.
When setting up a testing environment you should: use separate targets for development and production; use ref() macro, dbt seeds; prioritize critical data attributes; organize and structure tests; configure test severity and thresholds; use continuous integration; perform incremental testing, document the setup; review and update tests regularly; and finally – monitor test results.
Community-made testing packages, such as: dbt-utils, dbt-expectations, dbt-date, dq-tools, and dbt-meta-testing, provide additional tests, macros, and utilities that enhance dbt’s testing capabilities, ensuring data quality and consistency throughout data transformation processes.
What is proper project structure while using dbt for quality assurance? How the tests should look like? Read the article and find out!
Read more
Data quality refers to the condition or state of data in terms of its accuracy, consistency, completeness, reliability, and relevance. High-quality data is essential for making informed decisions, driving analytics, and developing effective strategies in various fields, including business, healthcare, and scientific research. There are six main dimensions of data quality:
Maintaining high quality of data often involves data profiling, data cleansing, validation, and monitoring, as well as establishing proper data governance and management practices to maintain high-quality data over time.
Data collection is widely acknowledged as essential for comprehending a company’s operations, identifying its vulnerabilities and areas for improvement, understanding consumer needs, discovering new avenues for expansion, enhancing service quality, and evaluating and managing risks. In the data lifecycle, it is crucial to maintain the quality of data, which involves ensuring that the data is precise, dependable, and meets the needs of stakeholders. Having data that is of high quality and reliable enables organizations to make informed decisions confidently.
While this example may seem quite dramatic, the value of quality management with respect to data systems is directly transferable to all kinds of businesses and organizations. Poor data quality can negatively impact the timeliness of data consumption and decision-making. This in turn can cause reduced revenue, missed opportunities, decreased consumer satisfaction, unnecessary costs, and more.
According to an IBM around $3.1 trillion of the USA’s GDP is lost due to bad data, and 1 in 3 business leaders doesn’t trust their own data. In a 2016 survey, it was shown that data scientists spend 60% of their time cleaning and organizing data. This process could and should be streamlined. It ought to be an inherent part of the system. This is where dbt might help.
Data Build Tool, otherwise known as dbt, is an open-source command-line tool that helps organizations transform and analyze their data. Using the dbt workflow allows users to modularize and centralize analytics code while providing data teams with the safety nets typical of software engineering workflows. To allow users to modularize their models and tests, dbt uses SQL in conjunction with Jinja. Jinja is a templating language, which dbt uses to turn your dbt project into a programming environment for SQL, giving you tools that aren’t normally available with SQL alone. Examples of what Jinja provides are:
In the context of quality management, dbt can help with data profiling, validation, and quality checks. It also provides an easy and semi-automatic way to document the data models. Lastly, through dbt, one can document the outcomes of some quality management activities, collecting the results and thus supplying more data on which the stakeholders can act.
In dbt tests are created as SELECT queries that aim to extract incorrect rows from tables and views. These queries are stored in the SQL files and can be categorized into two types: singular tests and generic tests. Singular tests are used to test a particular table or a set of tables. They can’t be easily reused but might be useful anyway. Generic tests are highly reusable, serving basically as test macros. For a test to be generic, it has to accept the model and column names as parameters. Additionally, generics can accept an infinite number of parameters as long as those parameters are strings, Booleans, integers, or lists of the mentioned types. This means that tests are reusable and can be constantly improved. Additionally, all tests can be tagged, which then allows running only tests with a specific tag if we want to.
It is possible to store test results in distinct tables, with each table holding the results for a single test. Whenever a test is run, its results overwrite the previous ones. But you can run queries on those tables and store the results by using dbt’s hooks. Hooks are macros that execute at the end of each run (there are other modes, but for now, this one is sufficient). By using the „on-run-end” hook, you can, for instance, loop through the executed tests, obtain row counts from each of them, and insert this information into a separate table with a timestamp. This data can now be easily utilized to generate a graph or table, providing actionable insights to stakeholders.
dbt has a self-documenting feature that allows for easy comprehension of the yaml configuration file by running the „dbt docs serve” command. The documentation can be accessed from a web browser, and it covers generic tests, models, snapshots, and all other dbt objects. In addition, users can include additional details in the YAML configuration, such as column names, column and model descriptions, owner information, and contact information. Users can also designate a model’s maturity or indicate if the source contains personally identifiable information. As previously noted, documentation of processes is a critical aspect of quality management. With dbt, this process is made easy, leaving no excuse for omitting it.
Generated documentation can also be used to track data lineage. By examining an object, you can observe all of its dependencies as well as the other objects that reference it. This data can be visualized in the form of a „lineage graph.” Lineage graphs are directed acyclic graphs that show a model’s or source’s entire lineage within a visual frame. This greatly helps in recognizing inefficiencies or possible issues further in the process when attempting to integrate changes.
Version control is a great technique that allows for tracking the history of changes and reverting mistakes. Thanks to version control systems (VCS) like Git, developers are free to collaborate and experiment using branches, knowing that their changes won’t break the currently working system. dbt can be easily version controlled because it uses yaml and SQL files for everything. All models, tests, macros, snapshots, and other dbt objects can be version controlled. This is one of the safety nets in the software developer workflow that dbt provides. Thanks to VCS, you can rest assured that code is not lost due to hardware failure, human error, or other unforeseen circumstances.
The importance of data quality for data analytics and engineering cannot be overstated. Ensuring data accuracy, completeness, consistency and validity is critical to making informed decisions based on reliable data, creating measurable value for the organization. Maintaining high data quality involves processes such as data profiling, validation, quality checks, and documentation. Data Build Tool (dbt), an open-source command-line tool, used for data transformation and analysis, can also greatly help with those tasks. dbt can assist in creating reusable tests, documenting test results, documenting data pipelines, tracking data lineage, and maintaining version control of everything inside a dbt project. By using dbt, organizations can streamline their quality management processes, enabling collaboration on data models while ensuring that data fulfills even the highest standards.
What is Data Quality, why is it so important and what tools can you use to ensure efficient transformation of data into value? Read the article and find out!
Read moreData Economy Congress as an event is the cross-sector meeting about the current data trends in business started with perfectly prepared trailer showing a kind of male robot which was supposed to embody the Artificial Intelligence. It was only surprising because in my mind AI was always female. Maybe it’s because of the movie „Ex Machine” or the first well-known robot known as „Sophia”. Perfect start of a well-designed conference. Nice structure of speeches and presentations intertwined with debates of branches’ specialists.
The first day of the event focused mostly on AI and its impact on our culture and Polish society. Introducing presentation hold by professor Dragan was tiled in a controversial way: „Will AI eat us” and it was not only a try to explain the way algorithms used by AI work in a quite simple way but also it gave some concerns about human’s skills to understand how the results are finally created. Closing slides gave attendees food for thought as AI can be as helpful as dangerous for humanity and it looks like it doesn’t depend only on us how it’s used anymore. The next point was a debate about cooperation between companies in terms of data exchange so that conclusions for different businesses could be drawn. The slogan cooperate or die sounds reasonable but in fact it’s quite a difficult topic as most of the organizations treat their data as their competitive advantage and sharing even aggregated information cause fear. The second part of the first day did shed the light on practical examples of AI usage in many organizations. While we as consumers have a feeling that the advised product/offer is targeted to us, we still think that this is done by marketing specialists and in fact it’s not. The most expected branch is healthcare which substantially impacts everyone’s lives. Even though it’s understandable that Artificial Intelligence could help us fight against civilizational diseases, Polish society is still afraid of giving consent to the use of personal medical records. However it seems that awareness and acceptance is increasing if a person gets enough explanation. Similarly to the problem with transplantation that we were experiencing. Social campaigns impacted this positively, which could also be the case for AI in medicine?
The final debate of the day was about the regulatory requirements that need to be introduced because of the European Data Governance Act and AI Act. The challenge that we face is not only connected with keeping the law up-to-date and predicting changes that will be needed while the AI is being developed but it also requires cooperation between regions like the USA or China. In case regulations are not introduced there, the unethical use of the AI and the advantage that could be taken from that could keep the AI development in Europe down and strengthen the sense of unfairness. On the other hand, we can’t stop working on AI development as from the medical point of view – we won’t be able to buy these technologies from others. The data for Europe and other parts of the world differ and this impacts results.
The second day of a congress didn’t let me down –it’s main concerns were data quality and reporting – topics that don’t sound as „sexy” as AI at first glance but are thoroughly practical for all businesses. The first debate entitled Customer experience personalization made participants discuss the approach to data privacy in customer profiling explaining their high attention to that topic. It appears that data-driven marketing in many branches is happening everyday but it’s technically done differently. Additionally, it was a very interesting organizational point raised, namely: ownership of data models. Although, AI and Data departments are structurally not in business organization units, this is the business that should be the owner of the predictive models.
The second block addressed the cyber-threats topic. There was a big discussion about fake news and the way they should be recognized in a real word. On the one hand, we know that the expanding velocity of truth is never as high as the expanding velocity of untruth messages. On the other hand, they should be caught so that we don’t make false decisions based on false premises. In the AI era, we will have more challenges with such challenges as the information, videos and artiles that could be generated by the AI. As such it is probably AI who (if I can treat it somehow human) should help recognizing them.
In the next sessions of the Congress, there were the topics around best practices in platform engineering covered. Very important debates about data locations, data quality, data mesh concept and ESG reporting in practice made me think companies’ perception changes. Not only did many of the leaders speak about the hybrid location as a thing of the future but they were also mentioning the pros and cons of company owned Data Centers, Colocations, Private Cloud and Public Cloud. On the other hand, the slogan: „garbage in, garbage out” increased its visibility among not only technical managers but also directors dealing purely with business.
We’re used to topics that should generate some income for companies but it definitely looks like sustainability also has its place. Probably mostly because of the changes in requirements concerning reporting of ESG. The discussion about standards and their common understanding makes me feel that an honest approach to the topic in all of the presented branches is crucial. Otherwise, the concern for sustainable development is artificial as only different categorization could cause variances in reporting and in that way possible commercial advantage. On the other hand, the statement made by one of the participants: „the real sustainability is now a competitive advantage over others” made me understand that for most of the companies, this interest is real and I hope this trend will be kept.
All in all, the Data Economy Congress in Warsaw was not only inspiring but also a well-prepared event. As BitHikers, we are surely going to attend it and join the discussions held next year, because looking at the technological solutions from business perspective is one of our core values and our technical knowledge and expertise can help in solving real challenges connected to AI, and its place in large scale businesses and society in general.
Thank you #DEC2023
The most important insights from recent Business and Data event in Warsaw which #BitHikers took part in - Data Economy Congress
Read moreIn the realm of data analytics, understanding and managing the complexities of data flow can be a challenging endeavour. Enter Data Flow Diagrams (DFD) – a tool often used by experienced data professionals. DFDs serve as visual roadmaps, illustrating the journey of data from its origin, through its processing stages, and onto its eventual use or storage. By offering a transparent view into flow of data and its architecture, these diagrams allow analysts to grasp the intricacies of data processes, making them indispensable in large-scale business analytics projects. Whether you are a novice seeking clarity or a seasoned analyst aiming for optimal data management, diving into this article will offer insight into the transformative power of DFDs and why they are a cornerstone in the world of data analytics.
Data flow diagrams can be categorized from the highest to the lowest level of abstraction, thus showing different levels of detail in data flow and transformation. Thanks to this, diagrams can be adapted to a given stakeholder and assumed objectives.
Context diagrams (Figure 1), the most general ones, present the entire data system. They indicate data sources and recipients as external entities that are connected by a transformation engine, i.e., a data processing centre between these entities.
The system-related processes are illustrated by the lower level DFDs, i.e., level 1 diagrams (Figure 2). This diagram type shows more detailed information distinguishing between individual data inputs, outputs, and repositories. Therefore, they can demonstrate the structure of the system and data flows between its depicted parts.
Then if it is required, decomposition of each system partition can be performed. As the result, the same external entities, further data transformations, stores and flows are obtained, however at the lower level (level 2 in Figure 3, level 3 diagram, etc.) giving increasingly detailed information.
In data flow diagram we can distinguish the following elements: external entities, data stores, data processes, and data flow, which are represented by different graphic symbols depending on the notation. Here we use the Gane and Sarson notation whose coding is shown in Table 1.
First one is tool, system, person or organization capable of generating or gathering data outside the analysed system. External entities can be where data is loaded from (data sources) and/or into (data destination). They are used at all levels of diagrams, starting from the context level and continuing downwards. An important requirement for such entities is that they indicate at least one flow of data that may enter or leave them.
The data store, the next element, is where the datasets are kept after loading and allows the data to be read multiple times. In other words, this is data at rest, waiting to be used. Data stores require at least one data flow, it can be incoming or outgoing.
Processes, on the other hand, are manual or automated activities that transform data into business-relevant results. They demand at least one incoming and one outgoing data flow.
Data flows illustrate the flux of data between the three above-mentioned elements and combine inputs and outputs of each data operation.
In BitPeak data flow diagrams are frequently used for portraying the data system in user friendly and understandable way for our Clients and coworkers. Such a technique makes it easier to exchange information about data model and its verification. With these diagrams, Business Analyst can clarify in an accessible and understandable way the logic and all the complexity of data flow to the Stakeholders involved ensuring alignment of business and data strategies.
We also use DFDs to determine the scope of the system and related to it elements, like user interfaces applied within, other systems and interfaces. These diagrams help in presenting relations with other systems (external entities) as well as between internal data process and stores. They can be useful for depicting boundaries of analysed system. Therefore, the required effort in project creation and valuation can be estimated. Additionally, it enables for decomposition of system at desired level to show adequate details of data flow. Deduplication of data elements and detection of their misapply can be reached with DFDs as they can easily track such objects and determine their function in the data flow. Diagrams also support the creation of documentation and the organization of knowledge about data and its flow.
However, there are few challenges with application of data flow diagrams, especially with big-scale systems. The larger the system, the more elements and relationships between them it contains. Therefore, respective diagrams are much larger and complex. This leads to rise of difficulty of understanding of DFD, and therefore data system by Stakeholders. Even with extensive experience in the data area, it is sometimes hard to grasp all the nuances of the analysed complex system with your own mind.
Another limitation is the fact that data operations alone provide small (but important) piece of information about business processes and stakeholders. Hence, a more complex analysis of the system using many techniques (e.g., business capability analysis, data mining, data modelling, functional decomposition, gap analysis, mind mapping, process analysis, risk analysis and management, SWOT analysis, workshops), including of course DFD, is required.
The next disadvantage Is not showing sequence of activities, but only depicting main data processes, so some important details are missed. However, thanks to that more general approach a clearer picture of system is received, which facilitates Stakeholders to follow the data flow from source through each data store to the final output.
Another challenge is plenty of notation methods used to create DFD as different symbols may cause confusion for the recipients of the documentation. The solution to this issue is very simple. All it takes is a conversation between the diagram creator with clients and project collaborators, specifying the requirements for the notation (in this article we have introduced Gane and Sarson notation), symbology used, level of detail, and information contained in the DFD.
Data Flow Diagrams (DFD) serve as a cornerstone in data analysis, providing a visual roadmap of data processes and flows between data entities. However, while they improve understanding and promote effective communication with stakeholders, challenges arise with system scale and varying notation methods. DFDs may not cover the full breadth of business processes, necessitating supplementary analysis techniques to avoid missing important elements. Nonetheless, their ability to simplify complex data systems and guide insightful business decisions underscores their significance in the data analytics landscape.
Good understanding between business and technology stakeholders can make or break data project. See how you can facilitate it through Data Flow Diagrams!
Read more
Artificial Intelligence has been a transformative force in various sectors, from healthcare to finance, and from transportation to entertainment and it does not seem to slow down with recent developments in generative AI. Its advent has brought about a paradigm shift in how we approach problem-solving and decision-making, enabling us to tackle complex tasks with unprecedented efficiency and precision.
However, as AI models become increasingly complex, they also become increasingly difficult when it comes to tracing its decision-making process in particular cases. This opacity, often referred to as the 'black box’ problem, poses a significant challenge. It’s like having a brilliant team member who consistently delivers excellent results but cannot explain how they arrive at their conclusions. This lack of transparency can lead to mistrust and apprehension, particularly when the decisions made by these AI models have significant real-world implications. If artificial intelligence is to be used in drafting new laws or as a support for healthcare providers, it must provide not only the answer but also the path it took to reach particular conclusion.
However all is not lost, as the 'black box’ problem has led to the emergence of Explainable AI (XAI) – a field dedicated to making AI decision-making transparent and understandable to humans. XAI seeks to open the 'black box’ and shed light on the inner workings of AI models. This is not just about satisfying intellectual curiosity; it’s about trust, accountability, and control. As we delegate more decisions to AI, we need to ensure that these decisions are not only accurate but also fair, unbiased, and transparent.
Explainable AI is a broad and multifaceted field, encompassing a range of techniques and approaches aimed at making AI systems more understandable to humans. At its core, XAI seeks to answer questions like: Why did the AI system make a particular decision in particular case? What factors did it take into consideration? On what basis did it make that decision? How confident is it in its decision? It is important to mention that XAI is not about understanding general mechanics of AI, as those are well understood by data scientists, but rather about the way AI connects concepts and weights particular parameters in a particular case.
When it comes to this aspect of explainability, there are two main approaches: interpretable models and post-hoc explanations.
Interpretable models are designed to be inherently explainable. They are typically simple models whose decision-making process is transparent and easy to understand. For instance, decision trees and linear regression models. In a decision tree, the decision-making process is represented as a tree structure, where each node represents a decision based on a particular feature, and each branch represents the outcome of that decision. This makes it easy to trace the path of decision-making and understand why the model made a particular decision.
However, interpretable models often trade-off some level of predictive power for interpretability. In other words, while they are easy to understand, they may not always provide the most accurate predictions. This is particularly true for complex tasks that involve high-dimensional data or non-linear relationships, which are often better handled by more complex models.
On the other hand, post-hoc explanations are used for more complicated systems like neural networks, which offer high predictive power but are not inherently interpretable. These models are often likened to 'black boxes’ because their decision-making process is hidden within layers of computations that are difficult to interpret.
Post-hoc explanation techniques aim to 'open’ these black boxes and provide insights into their decision-making process by generating explanations after the model has made a prediction or an answer. Hence the term 'post-hoc’. They provide insights into which features were most influential in making a particular decision, allowing us to understand why the model made particular response.
There are several post-hoc explanation techniques, each with its strengths and weaknesses. For instance, LIME (Local Interpretable Model-Agnostic Explanations) is a technique that explains the predictions of any classifier by approximating it locally with an interpretable model. On the other hand, SHAP (SHapley Additive exPlanations) is a unified measure of feature importance that assigns each feature an importance value for a particular prediction.
These techniques have been instrumental in making complex AI models more transparent and understandable. However, they are not without their challenges. For instance, they often require significant computational resources, and their results can sometimes be sensitive to small changes in the input data. Moreover, while they provide valuable insights into the decision-making process of AI models, they do not necessarily make the models themselves more interpretable.
However, as you will see below the research into the realm of Explainable AI (XAI) is ongoing, and variety of advanced modeling methods, services, and tools have been developed to enhance the interpretability and transparency of AI systems.
A study by Ma et al. (2023) explores the potential of voice-based conversational recommender systems (VCRSs) to revolutionize the way users interact with recommendation systems. These systems leverage natural language processing (NLP) and machine learning to generate human-like explanations of AI decisions, making AI more accessible and understandable to non-technical users. The researchers developed two VCRSs benchmark datasets in the e-commerce and movie domains and proposed potential solutions for building end-to-end VCRSs. The study aligns with the principles of explainable AI and AI for social good, utilizing technology’s potential to create a fair, sustainable, and just world. The corresponding open-source code can be found in the VCRS repository.
A study by Sharma et al. (2022) compares the viability of Tsetlin Machines (TMs) with other machine learning models prevalent in the field of recommendation systems. TMs are a type of interpretable machine learning model that uses simple, understandable rules to make predictions. The authors demonstrate that TMs can provide comparable performance to deep neural networks while offering superior interpretability and scalability. The corresponding open-source code can be found in the Tsetlin Machine repository.
A paper by Dhavala et al. (2020) introduces MLSquare, a Python framework designed to democratize AI by making it more accessible, affordable, and portable. The framework provides a single point of interface to a variety of machine learning solutions, facilitating the development and deployment of AI systems. The authors emphasize the importance of explainability, credibility, and fairness in democratizing AI, aligning with the principles of XAI. The corresponding open-source code can be found in the MLSquare repository.
It is worth mentioning that the above technologies represent just a fraction of the ongoing research and development efforts. As the field continues to evolve, we can expect to see even more innovative solutions aimed at enhancing the transparency and interpretability of AI systems, facilitating its use in more and more areas of our professional and private lives.
However, the technical and theoretical aspect of explainable AI is only part of the issue. After all the goal is not to create XAI just for the sake of intellectual curiosity, though that has value in itself, but also to create real-life applications and benefits. To illustrate, let’s look at a few case studies!
When it comes to artificial intelligence in the banking sector, JPMorgan Chase is using XAI to explain credit risk models to internal auditors and regulators. Credit risk models are complex AI models that predict the likelihood of a borrower defaulting on a loan. They play a crucial role in the bank’s decision-making process, influencing decisions on whether to approve a loan and at what interest rate. However, these models are typically 'black boxes’ that provide little insight into their decision-making process. By applying XAI techniques, JPMorgan Chase has been able to open these black boxes and provide clear, understandable explanations of their credit risk models. This has not only increased trust in these models and allowed for their optimization and adaptation to changing market environments but also helped the bank meet regulatory requirements.
In the field of healthcare, companies like PathAI are using XAI to provide interpretable AI-powered pathology analyses. Pathology involves the study of disease, and pathologists play a crucial role in diagnosing and treating a wide range of conditions. However, pathology is a complex field that requires a high level of expertise and experience as well as ability to parse and recall enormous amount of information. AI has the potential to assist pathologists by automating some of their tasks and improving the accuracy of their diagnoses. However, for doctors to trust and use these AI systems, they need to understand how they are making their diagnoses. By applying XAI techniques, PathAI has been able to provide clear, understandable explanations of their AI diagnoses, helping doctors understand and trust their AI systems. The key part here is healthcare professionals’ ability to check and verify answers provided by AI, which allows for easier and faster diagnostics while not compromising their accuracy and ability to assign responsibility for possible mistakes.
These case studies illustrate the power and potential of XAI. By making AI systems more transparent and understandable, XAI is not only building trust in AI but also enabling its more effective and responsible use. The Paper „Deep Learning in Business Analytics: A Clash of Expectations and Reality” by Marc Andreas Schmitt points out that one of the possible reasons for slower than expected adoption of Deep Learning in business analytics is lack of transparency and Black-Box problem, which makes it harder to build trust with both business users and stakeholders. XAI is an obvious way to solve this problem and open the way for faster and more efficient data transformations and data maturity in Enterprise Scale organizations.
The implications of XAI are far-reaching and have the potential to revolutionize how businesses operate. In sectors like finance and healthcare, where decision transparency is crucial, XAI can help build trust and meet regulatory requirements. By understanding how an AI model is making decisions, businesses can better manage risks and make more informed strategic decisions without exposing themselves to blindly trusting AI which can still make mistakes easily prevented through human oversight.
Moreover, XAI can also lead to improved model performance. By understanding how a model is making decisions, data scientists can identify and correct biases or errors in the model, leading to more accurate and fair predictions. For instance, a study by Carvalho et al. (2019) demonstrated that using XAI techniques to understand and refine a machine learning model led to a 5% improvement in prediction accuracy.
Beyond the aforementioned benefits, XAI can also foster innovation and drive business growth. By providing insights into how AI models make decisions, XAI can help businesses identify new opportunities and strategies. For instance, by understanding which features are most influential in a customer churn prediction model, a business can identify key areas for improving customer retention and develop targeted strategies accordingly.
Furthermore, XAI can also enhance collaboration between technical and non-technical teams within a business. By making AI understandable to non-technical stakeholders, XAI can facilitate more informed and inclusive discussions around AI strategy and implementation. This can lead to better decision-making and more effective use of AI across the business in general.
As we look towards the future, several emerging trends in XAI are poised to shape the landscape of AI transparency and interpretability. These trends are driven by ongoing research and development efforts, as well as the evolving needs and expectations of various stakeholders, including businesses, regulators, and end-users.
One significant trend is the development of hybrid models that combine the predictive power of complex models with the interpretability of simpler ones. These hybrid models aim to offer the best of both worlds: high predictive accuracy and interpretability. This approach is particularly promising for applications where both accuracy and transparency are critical, such as healthcare and finance. For instance, a study by Sajja et al. (2020) demonstrated the effectiveness of using XAI in the fashion retail industry to facilitate collaborative decision-making among stakeholders with competing goals.
Another exciting area of development is the use of natural language processing (NLP) to generate human-like explanations of AI decisions. By translating complex AI decisions into clear, understandable language, NLP can make AI even more accessible and understandable to non-technical users. This approach could democratize AI, enabling more people to leverage its benefits and contribute to its development. A study by Duell (2021) highlighted the potential of using XAI methods to support ML predictions and human-expert opinion in the context of high-dimensional electronic health records.
Moreover, as AI continues to evolve, we can expect to see new forms of explainability emerging. For instance, visual explainability, which uses visualizations to explain AI decisions, is an emerging field that could provide even more intuitive and accessible explanations of AI. This approach could be particularly effective for explaining AI decisions in fields like image recognition and computer vision, where visual cues play a crucial role.
One example of such is Grad-CAM, or Gradient-weighted Class Activation Mapping. A technique for making Convolutional Neural Networks (CNNs) more interpretable and transparent. It was proposed by Selvaraju et al. and has since been widely adopted in the field of Explainable AI.
Grad-CAM works by generating a heatmap for a given input image, highlighting the important regions that the CNN focuses on for a particular output class. This is achieved by calculating the gradient of the output class score with respect to the final convolutional layer activations. The resulting gradient weight map indicates the importance of each activation, which is then multiplied with the activation map to generate the Grad-CAM heatmap. This heatmap can then be upscaled and overlaid on the input image to provide a visual explanation of the CNN’s decision-making process.
The Grad-CAM process is based on several steps such as:
The Grad-CAM technique offers several key advantages as it operates as a post-hoc method, meaning it can be applied to any pre-trained CNN model without the need for retraining. Additionally, it can explain CNN predictions at different levels of granularity by using convolutional layers at different depths as well as highlight both class-discriminative and class-agnostic regions, providing a holistic understanding of the CNN’s reasoning process.
In the context of visual explainability, Grad-CAM represents a significant step forward. By highlighting the areas of an image that most influence a network’s decision, it provides valuable insights into how certain layers of the network learn and what features of the image influenced the decision.
However it is worth mentioning that as a study by Pi (2023) pointed out, the future of XAI is not just about technical advancements. It’s also about governance and security. As AI becomes increasingly integrated into our lives and societies, ensuring the transparency and accountability of AI systems will become a critical aspect of algorithmic governance. This will require collaborative engagement from all stakeholders, including the public sector, enterprises, and international organizations.
Explainable AI is a rapidly evolving field that holds the promise of making AI more transparent, trustworthy, and effective. As we continue to rely on AI for critical decisions, the importance of understanding these systems will only grow. Through advancements in XAI, we can look forward to a future where AI not only augments human decision-making but also does so in a way that we can understand and trust.
As we move forward, it’s crucial that we continue to prioritize explainability in AI. This is not just about meeting regulatory requirements or building trust; it’s about ensuring that we maintain control over AI and use it in a way that aligns with our values and goals. By making AI explainable, we can ensure that it serves us, rather than the other way around.
Perhaps the best way to prevent Skynet from annihilating human race is not another Sarah Connor, but understanding and modifying its decision-making process to make it less homicidal.
Dive into an article that tries to open the "black box" and unravel the complexities of AI, and see how we can make it understandable and transparent for through the Explainable AI approach.
Read moreSince 2016, Microsoft has strived to become an AI powerhouse on the global scale. The goal is to transform Azure into an artificial intelligence augmented machine with superlative capabilities. To this end, they partnered with OpenAI to build their infrastructure and democratize data. As of now, there are several promising results. Such as the infrastructure used by the OpenAI to train its breakthrough models, deployed in Azure to power category-defining AI products like GitHub Copilot, DALL·E 2, and ChatGPT. And Microsoft is not shy about gloating about their progress.
Recently, BitPeak representatives were invited to an event, titled “Azure and OpenAI: Partners in transforming the world with AI”. In this article we will share with you the key points of the Webinar, such as Microsoft strategy, established implementations and use cases, as well as a quick peak into the future of GPT-4.
So, if you are interested in AI, as you should be, you are in luck! Without further ado – let us dive in.
The hosts started strong and put emphasis on the necessity of investments in AI for companies that do not want to be left behind, as constant development creates pressure to progress or become uncompetitive. It was quite an obvious prelude for further promotion of Microsoft’s product, but the sentiment itself is not wrong. AI has come to the mainstream, with decently reliable results and cost-efficiency – and the world is riding on its wave.
In its 2022 report about AI, creatively titled “The state of AI in 2022—and a half decade in review” McKinsey supports this conclusion and gives their own insights about the future of artificial intelligence. Unfortunately for all the Luddites, the future with AI powered toasters and/or Skynet is confidently coming our way.
So, how does Microsoft prepare for the coming of our future computer overlords? The answer is simple:
The obvious Microsoft flagship is the ChatGPT which conquered the globe in lightning-fast time, reaching 100M users in just two months. In comparison, Facebook took 4.5 years to do the same. The chatbot won the minds and hearts through a combination of its ability to conduct nearly human-like conversations, provide code snippets and explanations, as well as very confidently state very incorrect information. And those are some very human competencies that not every person I know possesses.
But, jokes aside, why is ChatGPT so special and different from other chatbots? The concept itself is not new. However, as demonstrated during the webinar, you can ask it to create a meal plan for a particular family with concrete specifications such as portions, cooking style and nutrition. The bot will create (not paste!) such a plan for you and even provide a shopping list if asked. The list may be wrong the first time, but after some prodding you will get what you need and be ready to go to the nearest supermarket.
The example shows that not only does the AI have some real day-to-day uses, not only can it correct itself (or at least provide the second most probable answer based on its parameters), but also provide assistance in a broad range of topics with various capabilities. But, after knowing “why”, let us look closer at “how”.
The first part is its architecture. ChatGPT is a single model with multiple capabilities, often referred to as a „single model for multiple tasks”. This is the result of its underlying architecture and training methodology. Such an approach stands in contrast to the traditional solutions, which involve training separate models for each task. But how does it work exactly?
Transfer learning: ChatGPT leverages transfer learning, where it is pretrained on a large corpus of diverse text data, gaining a general understanding of language, facts, and reasoning abilities. This pretraining step enables the model to learn a wide range of features and patterns, which can be fine-tuned for specific tasks. The shared knowledge learned during pretraining allows the model to be flexible and adapt to various tasks without the need for individual task-specific models.
Zero-shot learning: Owing to its extensive pretraining, ChatGPT possesses the ability to perform zero-shot learning in which the model is trained on a set of labeled examples, but is then evaluated on a set of unseen examples that belong to new classes or concepts. This means it can handle tasks it has not been explicitly trained for, using only the knowledge acquired during pretraining. To achieve this, zero-shot learning relies on the use of semantic embeddings, which represent objects or concepts in a continuous vector space. By using these embeddings, the model can generalize from known classes to new classes based on their similarity in the vector space.
Few-shot learning: ChatGPT can also engage in few-shot learning, where it can learn to perform a new task with just a few examples. In this setting, the model is provided with examples in the form of a prompt, which helps it understand the task’s context and requirements. To achieve this, few-shot learning typically employs techniques like transfer learning, meta-learning, and episodic training. Transfer learning involves adapting a pre-trained model to a new task with limited data, while meta-learning involves training a model to learn how to learn new tasks quickly.
Thanks to this approach chatbot is more efficient when it comes to allocating resources, simpler to deploy, better at generalization and adaptation to new tasks, easier to maintain and able to find and use synergies between its capabilities. Why do other AI models either do not use this approach or are not as proficient in it?
The answer is simple – resources. ChatGPT benefits from an enormous amount of resources, both when it comes to infrastructure that supports its capabilities and the sourcing and parsing of training data.
But simple answers are usually not enough. Below are a few more tricks that the AI uses to answer questions ranging from Bar Exam tasks to trivia from the Eighties Show.
Safety: To increase safety, OpenAI employs Reinforcement Learning from Human Feedback (RLHF). During the fine-tuning process, an initial model is created using supervised fine-tuning with a dataset of conversations where human AI trainers provide responses. This dataset is then mixed with the InstructGPT dataset transformed into a dialog format. To create a reward model for reinforcement learning, AI trainers rank different model responses based on quality. The model is then fine-tuned using Proximal Policy Optimization, with this process iteratively repeated to improve safety.
Fine-tuning: Fine-tuning is achieved through a two-step process: pretraining and supervised fine-tuning. During pretraining, the model learns from a massive corpus of text, gaining a general understanding of language, facts, and reasoning abilities. In the supervised fine-tuning stage, custom datasets are created by OpenAI with the help of human AI trainers who engage in conversations and provide suitable responses. The model then fine-tunes its understanding by learning from these responses, improving its contextual understanding and coherence.
Scaling: Scaling is accomplished primarily by increasing the number of parameters in the model. ChatGPT in its newest iteration has billions of parameters that allow it to learn more complex patterns and relationships within the training data. The transformer architecture enables efficient scaling by leveraging parallelization and distributed computing, allowing the model to process vast amounts of data efficiently.
Reduced prompt bias: To reduce prompt bias, OpenAI explores techniques such as rule-based rewards, where biases in model-generated content are penalized. Another approach is to use counterfactual data augmentation, which involves creating variations of the same prompt and training the model on these diverse prompts to produce more consistent responses.
Transformer architecture: The transformer architecture, introduced by Vaswani et al. in 2017, is the foundation of GPT-4 and other state-of-the-art language models. Key features of this architecture include:
By leveraging these advanced features, the transformer architecture empowers ChatGPT to generate more contextually accurate, coherent, and human-like text compared to other AI models.
To establish and retain a dominant position in the AI tech-sphere, Microsoft has been actively pursuing strategic partnerships with leading research institutions, startups, and other technology companies. These alliances enable Microsoft to tap into external expertise, share knowledge, and jointly develop cutting-edge AI solutions, broadening their offer of AI-augmented services and tailoring them to their infrastructure. The most important partner is obviously OpenAI, which together with Microsoft develops four main models.
GPT series models, such as GPT-3 and GPT-4 are series of language models developed by OpenAI consisting of some of the largest and most powerful language models to date, with possibly up to 100 trillion parameters in the case of GPT-4 and respectable 175 billion in the case of GPT-3.
GPT-3 is capable of understanding and generating human-like text based on the input it receives. It can perform various tasks, including translation, summarization, question-answering, and even writing code, without the need for fine-tuning. GPT-3’s capabilities have opened up exciting possibilities in natural language processing and have garnered significant attention from the AI community opening it up to mainstream with obvious day-to-day uses.
Building on the success of GPT-3, OpenAI introduced GPT-3.5 and then GPT-4, with each new iteration bringing significant improvements. GPT-3.5 enhanced fine-tuning capabilities and context relevance, while GPT-4, surpassing all previous models, showcases superior complexity and performance. Leveraging the capabilities of GPT-3 like translation, summarization, and code writing, GPT-4 demonstrates heightened understanding and generation of human-like text, expanding the potential applications of AI in various sectors and daily life.
Codex is an AI model built on top of GPT-3, specifically designed to understand and generate code. It can interpret and respond to code-related prompts in natural language and can generate code snippets in various programming languages. The most notable application of Codex is GitHub Copilot, an AI-powered code completion tool developed by GitHub (a Microsoft subsidiary) in collaboration with OpenAI. Copilot assists developers by suggesting code completions, writing entire functions, and even recommending code snippets based on the context of the developer’s current work. Despite its recent legal troubles, it is no doubt a useful tool.
DALL-E is an AI model that combines the capabilities of GPT-3 with image generation techniques to create original images from textual descriptions. By inputting a text prompt, DALL-E can generate a wide array of creative and often surreal images, showcasing the model’s ability to understand the context of the prompt and generate relevant visual representations. DALL-E’s unique capabilities have implications for many creative industries, such as advertising, art, and entertainment, especially when it comes to lowering the entry threshold.
ChatGPT is a AI model fine-tuned specifically for generating conversational responses. It is designed to provide more coherent, context-aware, and human-like interactions in a chat-based environment. ChatGPT can be used for various applications, including customer support, virtual assistants, content generation, and more. By being more focused on conversation, ChatGPT aims to make AI-generated text more engaging, relevant, and useful in interactive scenarios. And while making jokes or understanding Norman McDonald’s humor may be beyond it (so far), the capability is still uncanny.
It is obvious that Microsoft decided to promote AI, seeing the potential to become a main facilitator and infrastructure provider, while also democratizing the whole process and fulfilling its mission of increasing productivity on a global scale. However, during the event it was strongly stated that the partnership with OpenAI, while productive and important, is only part of the range of services offered by Microsoft. The company uses its machine modeling muscles in a variety of ways, presented below, with both old services with AI augmentation and new propositions aimed at increasing productivity.
Now, with figures such as Elon Musk and Bill Gates cautioning against AI and its growth the question of ethics in research and development appears. And while it is rather improbable that ChatGPT, being just a weighed statistical model becomes Roko’s Basilisk – the dangers of automation, unethical data sourcing and increased dependence on quick and easy answers generated by ChatGPT – remain.
So what steps are taken during development of new generation of AI models to ensure that it does more good than bad and won’t go Skynet on the general populace?
Ethical principles: Microsoft has established a set of ethical principles that guide the development and deployment of AI. These principles include fairness, reliability and safety, privacy and security, inclusiveness, transparency, and accountability.
Bias detection and mitigation: Microsoft uses a combination of algorithms and human reviewers to detect and mitigate bias in its AI services. For example, it has developed tools that can identify and correct biased language in chatbots like ChatGPT.
Data privacy and security: Microsoft has strict policies and procedures in place to protect the privacy and security of user data. It also provides users with tools and settings to control how their data is used.
Explainability and transparency: Microsoft aims to make its AI services more explicable and transparent to users. It has developed tools like the AI Explainability 360 toolkit, which allows developers to understand and explain the decisions made by AI models.
Partnerships and collaborations: Microsoft collaborates with governments, NGOs, and academic institutions to ensure that its AI services are used for the social good. For example, it partners with organizations like UNICEF and the World Bank to develop AI solutions that address social and environmental challenges.
Responsible AI initiative: Microsoft has launched a Responsible AI initiative to promote the development and deployment of AI that is ethical, transparent, and trustworthy. The initiative includes a set of tools and resources that developers can use to build responsible AI solutions.
But all of those did not prevent the chatbot from being implicated in a civil libel case filed by Victorian Mayor Brian Hood who claims the AI chatbot falsely describes him as someone who served time in prison as a result of a foreign bribery scandal. Additionally, there are some questions about the regulations about data privacy that may be breached by ChatGPT, which resulted in it being banned in Italy.
The watchdog organization being the bad referred to „the lack of a notice to users and to all those involved whose data is gathered by OpenAI” and said there appears to be „no legal basis underpinning the massive collection and processing of personal data in order to 'train’ the algorithms on which the platform relies”. It is also telling that the AI researcher apologized and committed to working diligently and rebuilding violated trust.
So, while artificial intelligence presents enormous opportunities, and both Microsoft and OpenAI try to conduct their research in an ethical way, it is important to stay informed and watchful about potential dangers and opportunities.
To end the section about Microsoft’s strategy and development of AI products, the most important part must be mentioned – pricing.
The answer for the questions about using GPT for business is simple – tokenization
The prices itself can and probably will change, as demand stabilizes, but the “pay-as-you-go” model is promising and allows for great flexibility as well as somehow predictable costs. Additionally, there are few AI models to choose from, either focusing on “reasoning” ability or cutting costs.
All in all, Microsoft’s AI strategy and partnership with OpenAI have the potential to significantly shape the future of AI technology and its applications across various industries. By democratizing AI, integrating AI capabilities into its products, and fostering strategic collaborations, Microsoft is poised to remain at the forefront of the AI revolution, driving innovation and enabling unprecedented advancements in the field. Most importantly for the company, they want users to depend on their productivity increasing services and providers of AI-based solutions to depend on their infrastructure and processing power.
This is a natural extension of Microsoft business strategy, but differently than Azure or Power BI – their hegemony in the AI-sphere is as of now nearly uncontested. Even Google seems to be unable to find the right answer, perhaps because their own AI, Bard, has a habit of providing the wrong ones. For us, mere mortals, all is left to do is keep abreast of developments, hope that ethics prevail during the research and be prepared for a world run with or by AI.
How Microsoft acts to become the most important provider of AI backed services? Read and learn!
Read more
After the second part of the article series about Data Vault where we talked about data modelling and architecture, we return to you with quik look into naming conventions as well as the summary of the topic. It is great opportunity to learn something new, or just refresh your knowledge about Data Vault.
As we have already seen, the Data Vault is a multitude of tables with different structures and purposes. With hundreds of such objects in the warehouse, it is impossible to use them if we do not set the right naming rules.
Below is a sample set of prefixes for Data Vault objects:
Layer | Data Vault object | Name prefix |
RDV | Hub | H_ |
RDV | Satellite | S_ |
RDV | Multiactive satellite | SM_ |
RDV | Relational link | L_ |
RDV | Hierarchical link | LH_ |
RDV | Non-hierarchical link | LT |
BDV | Hub | BH_ |
BDV | Satellite | BS_ |
BDV | Multiactive satellite | BSM_ |
BDV | Relational link | BL_ |
BDV | Hierarchical link | BLH_ |
BDV | Non-hierarchical link | BLT |
Other | PIT | PIT_ |
Other | Bridge | BR_ |
Other | View | V_<DV_object_prefix> |
In addition to prefixes, it is worth standardizing the naming of related objects such as satellites around a common HUB and the naming of links. It is worth naming technical and business columns consistently. A dictionary of abbreviations and a dictionary of column prefixes and suffixes can be introduced.
If you’ve made it this far, you should already have a rough idea of what Data Vault is, how to create it, and what its advantages are. In my opinion, in order for the methodology to be used correctly it is also necessary to be aware of its disadvantages in order to prepare for their mitigation. For me, the fundamental disadvantage of Data Vault is the multiplicity of tables in the model and the difficulty in connecting them. Let’s say we want to write a cross-sectional query that retrieves data from three business hubs. Let’s say we need data from 2 satellites connected to each of these hubs (that’s already 9 tables). In addition, there are links between the hubs, and if there are satellites attached to the links, they also have to be included, which gives a total of (9+4) 13 tables that we have to involve.
This creates challenges in several areas:
Of course, each of these points can be addressed, but it requires additional work that one should be aware of.
The fragmentation of tables is, on the one hand, a disadvantage that I mentioned above, but on the other hand, it also has its advantages. For data warehouses with multiple consumers, many sources, and many critical processes, fragmentation helps to minimize the impact of any errors in data feeding. For example, we read a small dictionary from a CSV file and based on it, calculate a column in the Data Vault satellite. When this file does not appear or appears with an error, we will not feed only that one satellite in the data warehouse.
The rest of the data warehouse will work correctly, and the processes based on it. In the case of choosing a different modeling approach, where broad tables are created, a problem with one small element can cause a problem with feeding one of the most important data warehouse tables, delaying most critical processes. Fragmentation also makes data storage more efficient – we store data immediately after it appears. There are no situations where we wait for data from, for example, five sources, which we then combine in ETL and store. It is clear that in such an approach, ETL can only start after all the input data has appeared, so the writing is delayed by this waiting time, unlike in Data Vault.
Fragmentation also helps in developing a data warehouse in many independent teams and releasing such changes. Data Vault is very „agile” and greater gradation of data and feeding processes means we have fewer dependencies between teams. It looks completely different when we have critical and broad tables in the model and many teams that modify them. In such cases, conflicts are not difficult, and the effort required for integration and regression testing is much greater.
How to effectively manage a Data Vault model? I don’t want to give advice on when to create a new satellite and under what rules, because in my opinion it must be tailored to the company and how the data warehouse is to be developed. However, I would like to draw attention to the elements that must be addressed in order not to fail during the development of a Data Vault model consisting of hundreds of tables.
First of all, the production process should be described, which establishes the rules for developing the data warehouse, from the moment the data requirements appear to the implementation stage and then maintenance. I will not go into details here because this is a topic for a separate article, but I will only emphasize the fact that the model must be properly documented, that the rules for development (adding additional tables to the model) should be defined, that object and column naming should be consistent, and that a framework should be created to automate the feeding of DV objects (calculating keys, hdif, partitioning, etc.). It is also best for such a fragmented model to refer to something at a more generalized level. In the company, a high-level Corporate Data Model should be created, which the fragmented model must be consistent with (we always model down: CDM -> Data Vault Model).
The Data Vault model is a business-oriented approach to data, not source systems. Business concepts are usually constant, while IT systems live and change much more often. If we want to have a consistent model that does not change with the exchange of the IT system underneath, then Data Vault is the right choice. However, is it recommended for every organization? Definitely not. If you want to integrate several dozen or hundreds of data sources in the company, and if the company does not have dozens or hundreds of critical processes, then Data Vault is unecessary. The overhead required for a proper solution preparation can also be significant. The larger planned data warehouse is, the more certain the Return On Investment (ROI). ROI increases when:
So is Data Vault right for you? To answer your question you will need thorough understanding of your business needs and strategy, as well as knowledge about adventages and weaknesses od Data Vault. However, after reading our Data Vault series, you should be much better equipped to start answering the question.
This concludes the third and final part of our series of articles about Data Vault and its implementation. However, if you are curious about experts opinion and insights about data science, integration of data engineering solutions and synergizing technological and business strategy during data transformation – you are in luck!
Our experts create comperhensive and informative articles about the data analytic business. So tune in on our site and social media linked below to not miss valuable content.
And if you have additional questions about data – let’s talk about it!
What is Data Vault? How to implement it in your organization and how to harness its power in your organization? Take a look and learn!
Read moreAfter the first part of the article series about Data Vault where we introduced the concept and the basicis of its architecture, we return to you with more in-depth look into data modeling. We will analyze concepts such as Business keys (BKEYs), hash keys (HKEYs), Hash diff (HDIF) and more!
In contrast to traditional data warehouses, Data Vault does not generate artificial keys on its own, nor does it use concepts such as sequences or key tables. Instead, it relies on a carefully selected attribute from the source system, known as the Business Key (BKEY). Ideally, the BKEY should not change over time and be the same across all source systems where the data is generated. While this may not always be possible, it greatly simplifies passive model integration. Furthermore, in the context of GDPR requirements, it is not advisable to choose business keys that contain sensitive data as it can be challenging to mask such data when exposing the data warehouse.
Examples of BKEYs may include the VAT invoice number, the accounting attachment number, or the account number. However, finding a suitable BKEY may not be an easy task. One best practice is to check how the business retrieves data from source systems and which values are used when entering data into the source system. Typically, these values, as they are known to the business, are good candidates for BKEYs. Often, the same data is processed in multiple source systems. For instance, in an organization with several systems for processing tax documents (invoices, receipts), natural document numbers (receipt/invoice numbers) may be used in some, while an artificial key (attachment number) may be used in others. In some cases, a sequential document number and an equivalent natural number are also used. In such situations, using an integration matrix can help identify the appropriate BKEY.
As we can see from the matrix, there are several potential BKEY keys, but only the document number appears in the majority of the sources from which we retrieve document data. If we use a BKEY key based on the document number, the data in the Data Vault model will naturally integrate. However, what will we get for data from „System 2„? For this data, we need to design an appropriate same-as link (a Data Vault object) that will connect the same data. More on this in the later part of the article.
It is important that the same BKEY keys from different source systems are loaded in the same way. Even if we want to format such a key, for example, by adding a constant prefix, we should do it in the same way for data from all sources.
In the DV model, all joins are performed using a hash key. The hash key is the result of applying a hash function (such as MD5) to the BKEY value. The hash key is ideal for use as a distribution key for architectures with multiple data nodes and/or buckets. Through distribution, we can efficiently scale queries (insert and select) and limit data shuffling, as data with the same BKEY values are stored on the same node (having received the same HKEY).
In Data Vault objects that store historical data (SCD2), HDIF represents the next versions of a record. HDIF is calculated by computing a hash value on all the meaningful columns in the table.
Date and hour of record loading.
Indication that a record has been deleted. It is important to note that in Data Vault 2.0 it is not recommended to use validity periods (valid from – valid to) to maintain historical records. As this requires costly update operations that are not efficient, especially for real-time data. In addition, for some Big Data technologies, update operations may not be available, which further complicates the implementation of validity periods. Instead, Data Vault recommends an insert-only architecture based on technical columns such as LoadTime and DelFlag to indicate when a record has been deleted.
For Data Vault tables that receive data from multiple sources, the source column allows for additional partitioning (or sub-partitioning) to be established. Proper management of the physical structure of the table enables independent loading of data from multiple sources at the same time.
Different types of Data Vault objects have different sets of technical columns, which will be discussed further in the article.
In classic warehouses, there are often so-called key tables in which keys assigned to business objects on a one-off basis are stored. Loading processes read the key table and, based on this, assign artificial keys in the warehouse. There are also sequences based on which keys are assigned, and sometimes a GUID is used.
All these solutions require additional logic to be implemented so that the value of the keys can be assigned consistently in the warehouse model. Often, these additional algorithms also limit the scalability of the warehouse resource. Passive integration is the opposite of this approach. Passive integration involves calculating a key on the fly during a table feed based only on the business key. With a deterministic transformation (hash function on BKEY), we can do this consistently in any dimension, e.g:
The above is possible, but only if we choose the BKEY correctly, so the necessary effort should be made to make the choice optimal. We should consistently calculate it with the same algorithm for all HUB objects in the model. The exception can appear when we know that we have potential BKEYs in different formats in the source systems, but a simple transformation will make it consistent. It is important that this transformation is of the 'hard rule’ type.
For example:
In system 1 we have the key BKEY: „qwerty12345”
In system 2 we have the key BKEY: „QWERTY12345”
We know that business-wise they mean the same thing. In this case, we can apply a „hard rule” in the form of a LOWER or UPPER function to make the keys consistent.
Unfortunately, there are also situations where we have completely different BKEYs in different systems, for example:
In system 1 we have the key BKEY: „qwerty12345”
In system 2 we have the key BKEY: „7B9469F1-B181-400B-96F7-C0E8D3FB8EC0”
For such cases, we are forced to create so-called same-as links, which we will discuss later in this article.
Data Vault objects appear in the same form in both the RDV and BDV layers. The differences between them are only in the way the values in these objects are calculated (Hard rules and Soft rules). The objects of each layer should be distinguished at the level of naming convention and/or schema or database
HUB type objects
Hubs in the Data Vault warehouse are objects around which a grid of other related objects (satellites and links) is created. A Hub is a 'bag’ for business keys. A Hub cannot contain technical keys that the business does not understand, the keys must be unique. Examples of HUBs could be: customer, bill, document, employee, product, payment, etc.
We feed the Hubs with keys (BKEY) from the source systems, one BKEY can represent data from multiple source systems. We can use some rules to calculate BKEY but only those that meet the hard rules (usually UPPER, LOWER, TRIM). We never delete data from the HUB, if a record has disappeared from the source systems then its key should remain in the HUB. Even if the data is loaded into the hub in error, we do not need to delete unnecessary keys.
It stores business attributes. We can have satellites with history (SCD2) or without history (SCD0/SCD1). We create a new satellite when we want to separate some group of attributes. We can do this for a number of reasons:
a) we want to store data of the same business importance (e.g. address data) in one place
b) we want to separate fast-changing attributes into a separate satellite. Fast-changing attributes are those that change frequently causing duplication of records in the satellite. Examples of such attributes could be e.g. interest rate, account balance, accrued interest, etc.
c) we want to segregate attributes with sensitive data for which we will apply restrictive permission policies or GDPR rules.
d) we want to add a new system to the warehouse and create a new satellite for it
e) others that for some reason will be optimal for us
Data Vault is very flexible in this respect. However, be sure to document the model well.
Multiactive satellite – a specific type of a satellite where the key is not only BKEY but also a special multiactivity determinant (one of the substantive attributes). An example of such a satellite is a satellite storing address data where the multiactivity determinant is the type of address (correspondence, main, residential).
We have one BKEY (e.g. login in the application) and several addresses. We can successfully replace the multiactivity satellite with a regular one by adding a multiactivity determinant column to the hashkey calculation. My experience shows that it is better to limit the use of multiactivity satellites for reasons of model readability and reading efficiency.
Link objects come in several versions:
Relational link – represents relationships between two or more objects which can be powered by complex business logic. Relationships must be unique – this is achieved by generating a unique hash for the relationship which is calculated from the hashes of the records it links. A link does not contain business columns (the exception is an nonhistorized link).
If we want to show history then we need to attach a satellite with a timeline to the link (effectivity satellite). The performance satellite can also contain additional business columns describing relationships.
Hierarchical link – used to model parent-child relationships (e.g. organisational structure) This type of link can of course also store history. To achieve that – just add an efficiency satellite to the link.
Non-historicised link (also known as transactional links) – a link that may contain business attributes within it, or may be associated with a satellite which has these attributes. The important thing is that it stores information about events that have occurred and will never be changed (like a classic fact table). Examples of such data are: system logs, invoice postings that can only be changed/withdrawn with another posting (storno accounting), etc.
Link same as – allows you to tag different BKEY keys in the HUB table that essentially mean the same thing business-wise. I have mentioned this in previous chapters when describing the selection of the optimal BKEY. It is very important to note that this link only combines BKEY keys that business mean the same thing, we do not use the same as to register relationships other than mutually explicit relationships. We can use advanced algorithms to calculate often non-obvious links and record the results of the calculation in the link.
Links such as „same as” can be used in situations when we want to indicate often non-obvious business relationships, but also in very mundane situations. For example, when two systems have completely different business keys that represent the same thing, or when a key changes over time and we want to capture and record that change.
PIT facility – The Data Vault model is fragmented, as we have many subject satellites correlated to HUBs. Queries in the warehouse often involve several HUBs and satellites correlated with them. Selecting data from a specific point in time can be a challenge for the database. To improve read performance we use Point In Time (PIT) objects. A PIT table is something like a business index.
The important point is that we create PITs for specific business requirements. We define a set of source data (hubs, satellites), combine selected tables of hubs, links and satellites in such an arrangement as the business expects, e.g. for a selected moment in time (selected timeline or other business parameter). These are objects that we can reload and clean at any time, depending on the requirements of the recipient and the limitations of the hardware/system platform. The PIT is constructed from keys that refer to the hub and satellites so that we can retrieve data from these objects with a simple „inner join„.
A PIT facility can also refer to links instead of HUBs and satellites attached to a link.
BRIDGE object – works similarly to the PIT object with the difference being that it does not speed up access to data on a specific date but speeds up reading of a specific HKEY. Like PIT objects, BRIDGE objects are also created for the specific requirements of the data recipient. Bridge objects contain keys from multiple links and associated HUBs.
The raw Data Vault model is not an easy model to use, it is difficult to navigate without documentation and therefore should not be made widely available to end users. The PIT as well as the Bridge objects help the end-user to read the DataVault data efficiently but it is important to remember that they are not a replacement for the Information Delivery (Data Mart) layers. They should be considered more as a bridge and/or optimisation object to produce higher layers. Of course, creating a PIT/Bridge object also costs money, so this optimisation method is used where there are many potential customers.
This concludes the second part of our series of articles about Data Vault and its implementation. Next week, you will be able to read about naming convention. Additionally, you will be able to find the summary of the information provided so far! To make sure you will not miss the next part of the series, be sure to follow us on our social media linked below. And if you have additional questions about data – let’s talk about it!
What is Data Vault? How to implement it in your organization and how to harness its power in your organization? Take a look and learn!
Read more
Data Vault, compared to other modelling methods is relatively new. There are not many specialists with experience when it comes to data warehouses in this architecture. The lack of practical knowledge often results in solutions that only partially comply with the guidelines. This results in achieved results not fulfilling expectations and not supporting business strategy properly. Implementation and performance are especially problematic and require in-depth consideration.
But if you are curious about enormous potential of Data Vault as a Data Governance tool – you came to right place. Tomasz Dratwa, BitPeak Senior Data Engineer and Data Governance expert with several years of experience in implementing and developing Data Vaults decided to write down the most vital issues that need to be considered while building DV in your organization. Issues such as implementation of modelling from the architecture level to the physical fields in the warehouse. We are sure that they will help anyone who considers a warehouse in a Data Vault architecture.
The article is mostly for people who have some experience in dealing with databases and data warehouses before. It does not explain the basics of creating a data warehouse, modeling, foreign keys, or what SCD1 and SCD2 are. For those unfamiliar with the concepts, the article may be a challenging lecture. However, for those well-versed in dealing with databases and data warehouses, or just determined and able to access the google – this will most certainly be a very valuable lecture.
Data Vault is a set of rules/methodologies that allow for the comprehensive delivery of a modern, scalable data warehouse. Importantly, these methodologies are universal. For example, they allow for modeling both financial data warehouses where data is loaded on a daily basis, and where backward data corrections are important, as well as warehouses collecting user behavioral data loaded in micro-batches. Data Vault precisely defines the types of objects in which data is physically stored, how to connect them, and how to use them. Thanks to these rules, we can create a high-performance (in terms of reading and writing) fully scalable (in terms of computing power, space, and surprisingly, also manufacturing!) data warehouse. Proper use of Data Vault enables us to fully leverage the scaling capabilities of Cloud, Big Data, Appliance, RDBMS environments (in terms of space and computing power). Additionally, the structure of the model and its flexibility allows for parallel development of the data warehouse model by multiple teams simultaneously (e.g., in the Agile Nexus model).
The two logical layers of the integrated Data Vault model are:
Both layers can physically exist in one database schema, and it’s important to manage the naming convention of objects appropriately. An issue which I will explain later. The Information Delivery layer (Data Marts) should be built on top of the above layers in a way that corresponds to the business requirements. It doesn’t have to be in the Data Vault format, so I won’t focus on Information Delivery design in this article.
Currently, Data Vault is most popular in Scandinavian countries and the United States, but I believe it is a very good alternative to Kimball and Immon and will quickly gain popularity worldwide.
Data Vault is „Business Centric” data model, which follows the business relationships rather than the systems and technical data structure in the sources. The data is grouped into areas, of which the central points are the so-called Hub objects (which will be discussed later). The technical and business timelines are completely separated. We can have multiple timelines because the time attributes in Data Vault are ordinary attributes of the data warehouse and do not have to be technical fields. On the other hand, Data Vault ensures data retention in the format in which the source system produced it, without loss or unnecessary transformations. It seems impossible to reconcile, yet it can be done.
Data Vault is a single source of facts, but the information an often be multi-faceted. Variants are necessary, because the same data is often interpreted differently by different recipients, and all these interpretations are correct. Facts are data as it came from the source; Such data can be interpreted in many ways, and with time, new recipients may appear for whom calculated values are incomplete. With time, the algorithms used for calculations may also degrade. Data Vault is fully flexible and prepared for such cases.
Data Vault is based on three basic types of objects/tables:
As you can see, the Data Vault model is not simple. Therefore, it is recommended to establish the appropriate rules for its development and documentation during the planning phase. It is also important to start modeling from a higher level. The best practice is to build a CDM (Corporate Data Model) in the company, which is a set of business entities and dependencies that function in the enterprise. The Data Vault model should refer to the high-level CDM in its detailed structure. Additionally, it is worth defining naming conventions for objects and columns. It is also necessary to document the model (e.g. in the Enterprise Architect tool).
In this article, we will focus only on the portion of the architecture highlighted on the diagram. To this end I will explain what the RDV and BDV layers are, how to model them logically and physically, and how to approach data modeling in relation to the entire organization. We will also discuss all types of Data Vault objects, good and bad practices for creating business keys, naming conventions, explain what passive integration is, and discuss hard rules and soft rules. I will try to cover all the key aspects of Data Vault, understanding of which enables the correct implementation of the data warehouse.
A crucial aspect of a data warehouse is the storage and computation of facts and dimensions. To optimize this process, it’s very important to understand the differences between hard and soft rules transformations. Typically, the lower levels of any data warehouse store data in its least transformed state. This is due to practical considerations, as storing data in the form it was received in is crucial. Why? Because it allows us to use that data even after many years and calculate what we need at any given moment. On the other hand, some transformations are fully reversible and invariant over time, such as converting dates to the ISO format or converting decimal values from Decimal(14,2) to Decimal(18,4). These data transformations in Data Vault are called Hard Rules. Sometimes, we also consider irreversible transformations (for example trimming) as Hard Rules, but we must ensure that the data loss doesn’t have a business or technical impact. All other computations that involve column summation, data concatenation, dictionary-based calculations, or more complex algorithms fall under soft rule transformations. Data Vault clearly defines where we can apply specific transformations.
In logical terms, the Data Vault model is divided into two layers:
Raw Data Vault (RDV) – Which contains raw data, with solely hard rules allowed for calculations. Despite this, the RDV model is fully business-oriented, with objects such as Hubs, Links, and Satellites arranged according to how the business understands the data. Technical data layouts, as found in the source system, are not allowed in this layer. This is known as the „Source System Data Vault (SSDV)”, which provides no benefits, such as passive model integration, which will be discussed later. This layer stores a longer history of data according to the needs of the data consumers. It is also a good practice to standardize the source system data types in this layer, for example, by having uniform date and currency formats.
Business Data Vault (BDV) – which allows for any type of data transformation (both hard and soft rules) and arranges the data in a business-oriented manner. The source of data for this layer is always the RDV layer. The fundamental rule of Data Vault is that the BDV layer can always be reconstructed based on the RDV layer. If all objects in the BDV layer are deleted, a well-constructed Data Vault model should allow for its re-population.
Both layers are accessible to users of the data warehouse and their objects can be easily combined. It is recommended to store tables from both the RDV and BDV layers in the same database (or schema) and differentiate them with an appropriate naming convention.
This concludes the first part of our articles about Data Vault and its implementation. Next week, you will be able to read about data modelling. To make sure you will not miss the next part of the series, be sure to follow us on our social media linked below. And if you have additional questions about data – let’s talk about it!
What is Data Vault? How to implement it in your organization and how to harness its power in your organization? Take a look and learn!
Read moreAs Artificial Intelligence develops, the need for more and more complex models of machine learning and more efficient methods to deploy them arises. The will to stay ahead of the competition and the interest in the best achievable process automation require implemented methods to get increasingly effective. However, building a good model is not an easy task. Apart from all the effort associated with the collection and preparation of data, there is also a matter of proper algorithm configuration.
This configuration involves inter alia selecting appropriate hyperparameters – parameters which the model is not able to learn on its own from the provided data. An example of a hyperparameter is a number of neurons in one of the hidden layers of the neural network. The proper selection of hyperparameters requires a lot of expert knowledge and many experiments because every problem is unique to some extent. The trial and error method is usually not the most efficient, unfortunately. Therefore some ways to optimise the selection of hyperparameters for machine learning algorithms automatically have been developed in recent years.
The easiest approach to complete this task is grid search or random search. Grid search is based on testing every possible combination of specified hyperparameter values. Random search selects random values a specified number of times, as its name suggests. Both return the configuration of hyperparameters that got the most favourable result in the chosen error metric. Although these methods prove to be effective, they are not very efficient. Tested hyperparameter sets are chosen arbitrarily, so a large number of iterations is required to achieve satisfying results. Grid search is particularly troublesome since the number of possible configurations increases exponentially with the search space extension.
Grid search, random search and similar processes are computationally expensive. Training a single machine learning model can take a lot of time, therefore the optimisation of hyperparameters requiring hundreds of repetitions often proves impossible. In business situations, one can rarely spend indefinite time trying hundreds of hyperparameter configurations in search for the best one. The use of cross-validation only escalates the problem. That is why it is so important to keep the number of required iterations to a minimum. Therefore, there is a need for an algorithm, which will explore only the most promising points. This is exactly how Bayesian optimisation works. Before further explanation of the process, it is good to learn the theoretical basis of this method.
Imagine a situation when you see clouds outside the window before you go to work in the morning. We can expect it to rain during any day. On the other hand, we know that in our city there are many cloudy mornings, and yet the rain is quite rare. How certain can we be that this day will be rainy?
Such problems are related to conditional probability. This concept determines the probability that a certain event A will occur, provided that the event B has already occurred, i.e. P(A|B). In case of our cloudy morning, it can go as P(Rain| Clouds), i.e. the probability of precipitation provided the sky was cloudy in the morning. The calculation of such value may turn out to be very simple thanks to Bayes’ theorem.
This theorem presents how to express conditional probability using the probability of occurrence of individual events. In addition to P(A) and P(B), we need to know the probability of B occurring if A has occurred. Formally, the theorem can be written as:
This extremely simple equation is one of the foundations of mathematical statistics [1].
What does it mean? Having some knowledge of events A and B, we can determine the probability of A if we have just observed B. Coming back to the described problem, let’s assume that we had made some additional meteorological observations. It rains in our city only 6 times a month on average, while half of the days start cloudy. We also know that usually only 4 out of those 6 rainy days were foreshadowed by morning clouds. Therefore, we can calculate the probability of rain (P(Rain) = 6/30), cloudy morning (P(Clouds) = 1/2) and the probability that the rainy day began with clouds (P(Clouds|Rain) = 4/6). Basing on the formula from Bayes’ theorem we get:
The desired probability is 26.7%. This is a very simple example of using a priori knowledge (the right-hand part of the equation) to determine the probability of the occurrence of a particular phenomenon.
An interesting application of this theorem is a problem inspired by the popular Let’s Make A Deal quiz show in the United States. Let’s imagine a situation in which a participant of the game chooses one of three doors. Two of them conceal no prize, while the third hides a big bounty. The player chooses a door blindly. The presenter opens one of the doors that conceal no prize. Only two concealed doors remain. The participant is then offered an option: to stay at their initial choice, or to take a risk and change the doors. What strategy should the participant follow to increase their chances of winning?
Contrary to the intuition, the probability of winning by choosing each of the remaining doors is not 50%. To find an explanation for this, perhaps surprising, statement, one can use Bayes’ theorem once again. Let’s assume that there were doors A, B and C to choose from. The player chose the first one. The presenter uncovered C, showing that it didn’t conceal any prize. Let’s mark this event as (Hc), while (Wb) should determine the situation in which the prize is behind the doors not selected by the player (in this case B). We look for the probability that the prize is behind B, provided that the presenter has revealed C:
The prize can be concealed behind any of the three doors, so (P(Wb) = 1/3). The presenter reveals one of the doors not selected by the player, therefore (P(Hc) = 1/2). Note also that if the prize is located behind B, the presenter has no choice in revealing the contents of the remaining doors – he must reveal C. Hence (P(Hc|Wb) = 1). Substituting into the formula:
Likewise, the chance of winning if the player stays at the initial choice is 1 to 3. So the strategy of changing doors doubles the chance of winning! The problem has been described in the literature dozens of times and it is known as the Monty Hall paradox from the name of the presenter of the original edition of the quiz show [2].
As it is not difficult to guess, the Bayesian algorithm is based on the Bayes’ theorem. It attempts to estimate the optimised function using previously evaluated values. In the case of machine learning models, the domain of this function is the hyperparameter space, while the set of values is a certain error metric. Translating that directly into Bayes’ theorem, we are looking for an answer to the question what will the f function value be in the point xₙ, if we know its value in the points: x₁, …, xₙ₋₁.
To visualize the mechanism, we will optimise a simple function of one variable. The algorithm consists of two auxiliary functions. They are constructed in such a way, that in relation to the objective function f they are much less computationally expensive and easy to optimise using simple methods.
The first is a surrogate function, with the task of determining potential f values in the candidate points. For this purpose, regression based on the Gaussian processes is often used. On the basis of the known points, the probable area in which the function can progress is determined. Figure 1 shows how the surrogate function has estimated the function f with one variable after three iterations of the algorithm. The black points present the previously estimated values of f, while the blue line determines the mean of the possible progressions. The shaded area is the confidence interval, which indicates how sure the assessment at each point is. The wider the confidence interval, the lower the certainty of how f progresses at a given point. Note that the further away we are from the points we have already known, the greater the uncertainty.
The second necessary tool is the acquisition function. This function determines the point with the best potential, which will undergo an expensive evaluation. A popular choice, in an acquisition function, is the value of the expected improvement of f. This method takes into account both the estimated average and the uncertainty so that the algorithm is not afraid to „risk” searching for unknown areas. In this case, the greatest possible improvement can be expected at xₙ = -0.5, for which f will be calculated. The estimation of the surrogate function will be updated and the whole process will be repeated until a certain stop condition is reached. The progression of several such iterations is shown in Figure 3.
The actual progression of the optimised function with the optimum found is shown in Figure 4. The algorithm was able to find a global maximum of the function in just a few iterations, avoiding falling into the local optimum.
This is not a particularly demanding example, but it illustrates the mechanism of the Bayesian optimisation well. Its unquestionable advantage is a relatively small number of iterations required to achieve satisfactory results in comparison to other methods. In addition, this method works well in a situation where there are many local optima [3]. The disadvantage may be the relatively difficult implementation of the solution. However, dynamically developed open source libraries such as Spearmint [4], Hyperopt [5] or SMAC [6] are very helpful. Of course, the optimisation of hyperparameters is not the only application of the algorithm. It is successfully applied in such areas as recommendation systems, robotics and computer graphics [7].
[1] „What Is Bayes’ Theorem? A Friendly Introduction”, Probabilistic World, February 22, 2016. https://www.probabilisticworld.com/what-is-bayes-theorem/ (provided July 15, 2020).
[2] J. Rosenhouse, „The Monty Hall problem. The remarkable story of math’s most contentious brain teaser”, January. 2009.
[3] E. Brochu, V. M. Cora, i N. de Freitas, „A Tutorial on Bayesian Optimization of Expensive Cost Functions, with Application to Active User Modeling and Hierarchical Reinforcement Learning”, arXiv:1012.2599 [cs], December. 2010
[4] https://github.com/HIPS/Spearmint
[5] https://github.com/hyperopt/hyperopt
[6] https://github.com/automl/SMAC3
[7] B. Shahriari, K. Swersky, Z. Wang, R. P. Adams, i N. de Freitas, „Taking the Human Out of the Loop: A Review of Bayesian Optimization”, Proc. IEEE, t. 104, nr 1, s. 148–175, January 2016, doi: 10.1109/JPROC.2015.2494218.
How to enhance Artifical Intelligence? Learn how to use Bayes’ theorem to optimize your machine learning models with us!
Read moreData Factory is a powerful tool used in Data Engineers’ daily work in Azure cloud service. The code-free and user-friendly interface helps to clearly design data processes and improve Developer experience. It has many functionalities and features, which are constantly developed and enhanced by Microsoft.
The tool is mainly used to create, manage and monitor ETL (Extract-Transform-Load) pipelines which are the essence of the data engineering world. Therefore, I can confidently say that Data Factory has become the most integral tool in this field in Azure. But have you ever thought about the cost, that the service generates each time it is run? Have you ever done a deep dive into consumption run details, in order to investigate and explain the final price you have to pay each month for the tool?
Whether you have hundreds of long-running daily pipelines or use Data Factory for 10 minutes, once a week in your organization, it generates costs. Therefore, it is a good practice to know how to deal with it and create well-designed, cost-effective pipelines. In this article, you will find out how the small details can double your monthly invoice for Data Factory service. Azure is a pay-as-you-go service, which means that you pay only for what you actually used. However, the pricing details might overwhelm at first sight, and I hope the article will help you understand it more deeply. When you open official website (here or here) you can see that costs are divided into two parts: Data Pipeline and SQL Server Integration Services. In this article I will discuss only the Data Pipeline part, so let’s analyze it together.
First of all, it is important to realize that you are not only charged for executing pipelines, but the cost for Data Pipeline is calculated based on the following factors:
You are charged for data pipeline orchestration (activity run and activity execution) by integration runtime hours. Azure offers three different integration runtimes which provide the computing resources to execute the activities in pipelines. The below table presents the cost for each integration runtime.
Type | Azure Integration Runtime Price | Azure Managed VNET Integration Runtime Price | Self-Hosted Integration Runtime Price |
Orchestration | 1$ per 1 000 runs | 1$ per 1 000 runs | 1.5$ per 1 000 runs |
Orchestration refers to activity runs, trigger executions and debug runs. If you run 1000 activities using Azure Integration Runtime you are charged $1. The price seems to be low, but if you have a process that runs a lot of activities in loops many times a day, you could be surprised how much it could cost at the end of the month.
If you want to study existing pipelines in Data Factory, I recommend you to check the value in Data Factory/Monitoring/Metrics section by displaying charts Succeeded activity runs and Failed activity runs. The sum of these values is a total number of activity runs. The below picture presents how you can check the statistics for Data Factory instance for last 24 hours.
As you can see in the above example, the pipelines are executed every 3 hours and the total number succeeded activity runs is 8320. How much does it cost? Let’s calculate:
Daily price: 8320/1000 * $1 = $8.32
Monthly price: 8320/1000 * $1 * 30 days = $249.6
Every pipeline execution generates cost. Pipeline activity is defined as an activity which is executed on integration runtime. The below table presents the pricing of execution Pipeline Activity and External Pipeline Activity. As demonstrated in the below table, the price is calculated based on the time of execution and the type of integration runtime.
Type | Azure Integration Runtime Price | Azure Managed VNET Integration Runtime Price | Self-Hosted Integration Runtime Price |
Pipeline Activity | $0.005/hour | $1/hour | $0.10/hour |
External Pipeline Activity | $0.00025/hour | $1/hour | $0.0001/hour |
Depending on the type of activity that is executed in Data Factory, the price is different, as illustrated in Pipeline Activity and External Pipeline Activity sections in the table above. Pipeline Activities use computing configured and deployed by Data Factory, but External Pipeline Activities use computing configured and deployed externally to Data Factory. In order to show which activity belongs where, I prepared the below table.
Pipeline Activities | External Pipeline Activities |
Append Variable, Copy Data, Data Flow, Delete, Execute Pipeline, Execute SSIS Package, Filter, For Each, Get Metadata, If Condition, Lookup, Set Variable, Switch, Until, Validation, Wait, Web Hook | Web Activity, Stored Procedure, HD Insight Streaming, HD Insight Spark, HD Insight Pig, HD Insight MapReduce, HD Insight Hive, U-SQL (Data Lake Analytics), Databricks Python, Databricks Jar, Databricks Notebook, Custom (Azure Batch), Azure ML, Execute Pipeline, Azure ML Batch Execution, Azure ML Update Resource, Azure Function, Azure Data Explorer Command |
While executing pipelines, you need you know that execution time for all activities is prorated by minutes and rounded up. Therefore, if the accurate execution time for your pipeline run is 20 seconds, you will be charged for 1 minute. You can notice that in the activity output details in the billingReference section. The below pictures present an example of executing Copy Data activity.
The section billingReference in output details of execution of the activity holds information like meterType, duration, unit. The pipeline was executed on self-hosted integration runtime and lasted 1/60 min = 0.016666666666666666 hour, although the time of execution was 20 seconds.
It was really surprising for me, that Azure charges for each inactive pipeline which has no associated trigger or zero runs within one month. The fee for it is $0.80 per month for every pipeline, so it is crucial to delete unused pipelines from Data Factory especially when you deal with hundreds of pipelines. If you have 100 unused pipelines in your project, the monthly fee is $80 and the yearly cost is $960.
Copy Data Activity is one of the options in Data Factory. You can use it to move the data from one place to another. It is important to know that in Settings you can change the default Auto value to 2. By doing so, you can decrease the data integration unit to a minimum, if you copy small tables. In general, the value of units can be in the range of 2-256 and Microsoft has recently implemented a new feature for Auto option. When you choose Auto, it means that Data Factory dynamically applies the optimal DIU setting based on your source-sink pair and data pattern.
The below table presents the cost of consumption of one DIU per hour for different types of integration runtime.
Type | Azure Integration Runtime Price | Azure Managed VNET Integration Runtime Price | Self-Hosted Integration Runtime Price |
Copy Data Activity | $0.25/DIU-hour | $0.25/DIU-hour | $0.10/hour |
Let’s estimate cost of a pipeline that has only Copy Data Activity.
Example:
If Copy Data Activity lasts 48 seconds, the copy duration time is rounded up to 1 minute, so the cost is equal to:
1 minute * 4 DIUs * $0.25 = 0.0167 hours * 4DIUs * $0.25 = $0.0167
As you can see the price $0.0167 seems to be low, but let’s consider it more deeply. If you execute the pipeline for 100 tables every day, the monthly cost is equal to:
$0.0167 * 100 tables *30 days = $50.1
If you execute the pipeline for 100 tables every single hour, the monthly cost is equal to:
$0.0167 * 100 tables * 30 days * 24 hours = $1,202.4
The most crucial part of creating the pipeline solution is to keep in mind that even if you handle small tables, but do it very often, it could dramatically increase the total cost of the execution. If it is feasible, I recommend preparing the data upfront and using one large file instead. You can just code a simple Python script.
The next factor that could be relevant in regard to pricing is Bandwidth. If you want to transfer the data between Azure data centers or move in or out the data of Azure data centers you can be additionally charged. Generally, moving the data within the same region and inbound data transfer is free, but the situation could be different in other cases. The price depends on the region, internet Egress and differs for Intra-continental or Inter-continental data transfer.
For example, if you transfer 1000 GB data between regions within Europe, the price is $20, but in South America it is $160. When it is necessary to move 1000 GB from Europe to other continents the price is $50, but from Asia to other continents it’s $80. Therefore, think twice before you decide where to locate your data and how often you will have to transfer it. As you notice, there are many factors contributing to the bandwidth price. You can find the whole price list in Azure documentation.
Data Flow is a powerful tool in ETL process in Data Factory. You can not only copy the data from one place to another but also perform many transformations, as well as partitioning. Data Flows are executed as activities that use scale-out Apache Spark clusters. The minimum cluster size to run a Data Flow is 8 vCores. You are charged for cluster execution and debugging time per vCore-hour. The below table presents Data Flow cost by cluster type.
Type | Price |
General Purpose | $0.268 per vCore-hour |
Memory Optimized | $0.345 per vCore-hour |
It is recommended to create your own Azure Integration Runtimes with a defined region, Compute Type, Core Counts and Time To Live feature. What is really interesting, is that you can dynamically adjust the Core Count and Compute Type properties by sizing the incoming source dataset data. You can do it simply by using activities such as Lookup and Get Metadata. It could be a useful solution when you cope with different dataset sizes of your data.
To sum up, in terms of Data Flows in general you are charged only for cluster execution and debugging time per vCore-hour, so it is significant to configure these parameters optimally. If you want to use one basic cluster (general purpose) for one hour and use a minimum number of Core Count, the total price of execution is equal to:
$0.268 * 8 vCores * 1 hour = $2,144
The monthly price is equal to:
$0.268 * 8 vCores * 30 days * 1hour = $64.32
There are four bottlenecks that depend on total execution time of Data Flow:
I want to focus on the first factor: cluster start-up time. It is a time period that is needed to spin up an Apache Spark cluster, which takes approximately 3-5 minutes. By default, every data flow spins up a new Spark cluster, based on the Azure Integration Runtime configuration (cluster size etc.). Therefore, if you execute 10 Data Flows in a loop each time, a new cluster is spun up, ultimately it can last 30-50 minutes just for start-up clusters.
In order to decrease cluster start-up time, you can enable Time To Live option. The feature keeps a cluster alive for a certain period of time after its execution completes. So, in our example each Data Flow will reuse the existing cluster – it starts only once, and it takes 3-5 minutes instead of 30-50 minutes. Let’s assume that the cluster start-up lasts 4 minutes.
| Scenario 1 – Estimated time of executing 10 Data Flows without Time To Live | Scenario 2 – Estimated time of executing 10 Data Flows with Time To Live |
Cluster start-up time | 40 min | 4 min (+ 10 min Time to Live) |
Reading from source | 10 min | 10 min |
Transformation time | 10 min | 10 min |
Writing to sink | 10 min | 10 min |
The table above presents two scenarios of execution 10 Data Flows in one pipeline, but the second option has Time To Live feature that lasts 10 minutes.
Cost of executing the pipeline in scenario 1:
70 mins/60 * $0.268 * 8 vCores = $2.5
Cost of executing the pipeline in scenario 2:
44mins/60 * $0.268 * 8 vCores = $1.57
It easy to see that the price in scenario 1 is much higher than in scenario 2.
The most crucial part of using Time to Live option is the way of executing the pipelines. It is highly recommended to use Time To Live only when pipelines contain multiple sequential Data Flows. Only one job can run on a single cluster at a time. When one Data Flow finishes, the second one starts. If you execute Data Flows in a parallel way, then only one Data Flow will use the live cluster and others will spin up their own clusters.
Moreover, each of them will generate extra cost from Time To Live feature, because clusters will wait unused for a certain period of time when they finish. In consequence, the cost could be higher than without Time To Live feature. In addition, before implementing the solution make sure if Quick Re-use option is turned on in integration runtime configuration. It allows to reuse a live cluster for many Data Flows.
The next actions that generate cost are the „read”, „write” and „monitoring” options. The below table presents the pricing.
Type | Price |
Read/Write | $0.50 per 50 000 modified/referenced entities |
Monitoring | $0.25 per 50 000 run records retrieved |
Read/write operations for Azure Data Factory entities include „create„, „read„, „update„, and „delete„. Entities include datasets, linked services, pipelines, integration runtime, and triggers. Monitoring operations include get and list for pipeline, activity, trigger, and debug runs. As you can see, every action in the data pipeline generates cost, but this factor is the least painful one when it comes to pricing, because 50 000 is really a huge number.
I would like to present you one feature that could be helpful in finding bottlenecks in your existing solution in Data Factory. First of all, every executed pipeline is logged in Monitor section in Data Factory tool. Logs contain a data of every step of the ETL process, including pipeline run consumption details, but there they are stored for only 45 days in Monitor. Nevertheless, it is feasible to calculate an estimated price of Pipeline orchestration and Pipeline execution.
I found PowerShell code on Microsoft community website that generates aggregated data of pipelines run consumption within one resource group for defined time range. I strongly believe that the code can be useful for costs estimation of your existing pipelines. It is worth mentioning that this method has some limitations and for example it doesn’t contain information about consumption of Time To Live in Data Flows. In the picture below you can see this information in the red box.
I hope you found this article helpful in furthering your understanding of pricing details and the features that could be significant in your solutions. Microsoft is still improving Data Factory and while preparing this paper I needed to change two paragraphs due to the changes in Azure documentation. For example, from January 2022, you will no longer need to manually specify Quick Re-use in Data Flows when you create an integration runtime and that is great information. I found a funny quote that could describe Azure pricing in general: You don’t pay for Azure services; you only pay for things you forget to turn off – or in this case – “turn on”.
See how to optimize the costs of using Azure Data Factory!
Read moreSitting in a cozy café in your favorite t-shirt, with one click you change into a shirt and put on a jacket. You can start a conference with your future client. Such perspective is becoming more and more real, and closer than ever, due to concept of Digital Fashion.
With the development of new technologies, especially 3D graphics (rendering, 3D models and fabric physics), the term is becoming increasingly popular. And what is Digital Fashion really? It is simply digital clothing – a virtual representation of clothing created using 3D software and then „superimposed” on a virtual human model.
Digital Fashion seems to be the next step in the development of the powerful e-commerce and fashion markets. Online stores started with descriptions and photos; now 360° product animations have become the norm, and digitally created models’ faces and bodies are increasingly being used for promotional graphics. The time for virtual fitting rooms and maybe even our own virtual wardrobes is coming. Actually, this (r)evolution has already taken its first steps. Let us just look at AR app projects of brands such as Nike (2019) or the collaboration of Italian fashion house Gucci with Snapchat (2020).
Where did the need for this type of solution come from? The main, but not the only, factors giving rise to this type of application are:
On-line work and social relations – more and more events are moving or taking place simultaneously in the virtual world. The same applies to professions and even social gatherings. Remote working „via webcam” is no longer the domain of the IT industry, but increasingly appears in the entire sectors of the economy.
Environmental consciousness — digital clothes and accessories do not require farmland or animal husbandry for fabric and leather, as well as 93 billion cubic meters of water to produce textiles, laundry detergents, or global distribution routes. Designed once anywhere in the world, they can be globally available in no time.
The rapid increase in the popularity of items that do not exist in the real world – NFTs (non-fungible tokens) and people adopting digital alter egos.
The new generations are natives of technology. They largely communicate, and thus express themselves, in the virtual world. A perfect example of this trend is the success of fashion house Balenciaga’s campaign done in cooperation with the game Fortnite. Digital-to-Physical Partnerships will become more and more common.
Above, I have only outlined the emerging niche of Digital Fashion. It is also worth mentioning Polish achievements in this field – those interested may refer to the VOGUE article on the Nueno digital clothing brand and the article on homodigital.pl. Personally, I am extremely curious what virtual reality will bring to the e-commerce and fashion market in the coming years.
The rapid development of the Digital Fashion niche observed in recent years gives us huge, still largely undiscovered opportunities for the development of new products and services in this area. From designers specializing only in Digital Fashion, through professionals selecting textures for virtual fabrics, to programmers responsible for the unique physics of clothes. Personally, my favorite option would probably be to turn off gravity – you are sitting safely in a chair, and the shirt you’re wearing is acting like you’re in outer space. So naturally, space is created for apps that showcase emerging products and for marketplaces where customers will be able to view and purchase them.
For the purpose of this article, we will take on the challenge of creating just such a solution – an AR app connected to a digital clothing marketplace. The application will give the user the to create their own virtual styling, and clothing brands, as well as related brands, to officially sell their products and NFT.
In theory, the operation is very simple – the application collects data about the user’s posture from the camera image, then processes it in real time using a library for human pose estimation (technology: OpenCV + Python). The collected data is actually just points in 3D space. They are transferred to the 3D engine, in which a virtual model of the User is created. The 3D model of the character itself is invisible, but interacts with visible clothes and/or accessories (technology: Blender 3D + Python). Ultimately, the user sees himself with the digital clothing superimposed.
At this point, it is worth clarifying two terms:
POSE ESTIMATION — pose estimation is a computer vision technique that predicts movements and tracks the location of a person. We can also think of pose estimation as the problem of determining the position and orientation of a camera relative to an object. This is usually done by identifying, locating and tracking a number of key points on a person, such as the wrist, elbow or knee.
RIGGING — (skeletal animation) means equipping a 3D model of a human, animal or other character with jointed limbs and virtual bones.These form a skeleton inside the model, which makes it much easier and more efficient for the animator to maneuver – movements of the bones affect the movement of the 3D model.
The exchange of information between the program making the pose estimation and the skeleton inside the human model is the basis of the created application. Data packets about the position of characteristic points on the body, which are x, y, z parameters in space, will be connected with the same points in rigging of the 3D model of the figure.
The proposed solution does not go in the direction of a virtual avatar (i.e. it does not position itself as a replacement for a person’s image). We are interested in the environment around the person, in the surroundings – clothes, accessories, interiors, etc. – what is around is already a product. Following the proverb „closer to the body than the shirt”, the closest and always fashionable product are clothes – hence we will strongly focus on this segment of the market.
The question arises – what if the user wants to change their eye color? From there it’s close to swapping your hand for that of the Terminator after the fight in the final scene. I identify such needs as very interesting (e.g. in Messenger filters), but infantile. I would describe the proposed solution as a place of man + product, rather than man + visual modification of man. This is intended to imply an image of greater maturity, professionalism and brand awareness. In practice, it is meant to be a place where existing brands can sell products right away. The product focus is also meant to clearly differentiate this solution from the filters familiar from TikTok/Instagram, or animated emoticons on iOS.
Just how fresh and hot the topic of digital clothing, and the entire emerging market associated with it is, is indicated by the huge interest generated by the Connect 2021 conference, during which the CEO of Facebook, or, for some, META, presented the Metaverse (’meta’- beyond, and 'universum’- world). This is the concept of a new internet combining the 'internet of things’ with the 'internet of people’. Mark Zuckerberg explained in an interview with The Verge that the Metaverse is „an embodied internet where instead of just viewing content – you are in it”. The author of the term itself is Neal Stephenson, who used it nearly thirty years ago in his cyberpunk book Snow Crash. In it, he describes the story of people living simultaneously in two realities – real and virtual.
The question is not „will it happen?” but rather „when and how it will happen?” As augmented, and virtual reality technologies become increasingly present in our lives, the world that now surrounds us on a daily basis will migrate into the Metaverse. Offices, pubs, gyms, flats are all now our mundane lives and will also be present in digital life. At the center, however, will always be people and their experiences. But what would interactions with others be like without the right attire? A „burning” t-shirt of your favorite band at a virtual concert; a waterfall dress during a New Year’s Eve meta-ball, or a golden shirt at a business meeting summarizing a successful project – although it sounds like science-fiction, this series of articles is an attempt to respond to such needs.
The evolution of the e-commerce market towards Digital Fashion has already begun. This is possible thanks to the dynamic development of technologies such as Pose Estimation, 3D graphics, and hundreds of other smaller, but very important, innovations appearing every day. In this article, we’ve given an overview of what digital clothing is and the opportunities it presents – for software developers on the one hand, and designers and graphic designers on the other.
In the future articles we will focus on technical issues related to the created application and market. Those interested can count on a large dose of code in Python associated with Pose Estimation and Blender 3D. There will also be plenty of news related to Digital Fashion and Metaverse.
Sitting in a cozy café in your favorite t-shirt, with one click you change into a shirt and put on a jacket.
Read moreAWS Glue is an arsenal of possibilities for data engineers to create ETL processes with Amazon resources. It supports a setup of calculating units where jobs can be in the form of Python or Spark scripts made from scratch or using AWS Glue Studio with an interactive visual designer. The designer has a simple interface and comes up with helpful set of ready to use transformations. Still, it also presents some limitation and problems.
The visual designer automatically generates a script for every added transformation. This script can be modified, however, any change to it will block the possibility for further visual development as user code cannot be translated into visual transformations.
Currently there are 15 available transformations, like Select Fields, Join, or Filter. Those basic operations cover up most of typical data operations, yet there is always a need for more complex calculations. In those situations, SQL and Custom transformations come to the rescue. First one extends the job’s capabilities only to SQL functions. Second one allows to create a new transformation with user made Python function that can only accept one parameter and always need to return DynamicFrameCollection.
If there is a need to extend a job with additional parameters they need to be added in the job’s configuration, yet they are also needed to be added manually to the script. If a developer builds the job with visual templates, it makes them impossible to do the development further in the visual designer, as a proper visual operation to add jobs’ parameters into script is not implemented.
Some transformations, like SelectFields, do not handle empty datasets in a proper manner. If empty dataset needs to be processed, those transformations will return an empty object without headers. This in turn will lead to an error in the next step, if any processing is applied on the indicated columns.
There are several problems with the web interface itself, i.e., a significant amount of used visual transformation leads to a complete slowdown of the designer, or if someone wants to change the data type for only one column in ApplyMapping with selection menu, this sometimes causes unexpected changes in all other columns.
Data preview is a great addition to AWS Glue Studio as it allows to observe how parts of data are processed through every transformation. However, if there is any error in a job, it prints a general error message and restarts itself to print the same message on and on. This does not allow to really validate the error, which sometimes forces you to stop viewing the Data preview and run the job in standard mode.
AWS Glue is an arsenal of possibilities for data engineers to create ETL processes with Amazon resources.
Read moreAWS Glue is, amongst other AWS services, a great choice for a Big Data project. Alone or even with other services, like AWS Step Function and AWS EventBridge, it may help create a fully operational system for data analysis and reporting. The service provides ETL functionalities, facilitates integration with different data sources and allows a flexible approach to development.
In the following paragraphs I present a review of AWS Glue features and its functionalities based on a real example of integration with external databases and loading data form there to S3 buckets. Whole purpose of this exercise is to present technical side of the service using a practical case and building a simple solution step by step.
In the reviewed case, the data source is a PostgreSQL database which is an external resource from AWS. It stores few tabular datasets that are supposed to be moved to Amazon S3. Someone could create a connection to scan this database directly In a form of a script, but here we can use AWS Glue Connections. It allows to create a static connection to databases which stores connection’s definition, the chosen user and its password. It delivers a possibility to connect external databases, Amazon RDS, Amazon Redshift, MongoDB and others.
Based on the established connection in AWS Glue, it is possible to scan databases to know what tables are available there. Developers can use AWS Glue Crawlers which may analyse whole databases model for a chosen database schema to create an internal representation of tables. A Crawler can be run manually or based on a schedule to scan one or more data sources. A successful scan of Crawler creates metadata in Data Catalog for Databases and Tables.
Databases in AWS Glue serve a purpose of containers for inferred Tables. Tables are just metadata and they reference actual data in an external source, i.e., their data are not saved in Amazon storage. In a situation where inferred Tables are created with Crawler scanning internal Amazon resources, those Tables would also act only as references. This means that deleting Tables in AWS Glue would only lead to deletion of metadata in Data Catalog, but not to deletion of physical resources on external databases or S3. What developers must also remember is that Tables from external resources are not available for ad-hoc queries using Amazon Athena, even though scanned Databases exists in Amazon Athena.
AWS Glue lets developers create Spark or simple Python jobs, where jobs’ settings can be modified to select type of workers, number of workers, timeouts, concurrency, additional libraries, job parameters and so on. Developers may create a job by writing and passing scripts using Amazon platform or using recent feature in AWS Glue Studio to create jobs with a visual designer.
Continuing with the case study, in the above picture there is a visually created job that would import data from PostgreSQL databases into S3 bucket. In this simple example, there are only three operations used (left side of the picture): Data source, Transform and Data target. Those operations and additional other built-in transformations simplify the process of creating Glue jobs. First operation directly creates a data frame from an external table by simply indicating Database and Table created in the previous steps. Then, by “filter” transformation, only specific data are saved into S3 bucket with the last operation.
All those three steps can be done manually just by the means of passing parameters in the visual designer. Moreover, visual transformations will generate a ready to run script (right side of the picture). This script can be modified, but that irreversibly switches off a possibility of further modification using the visual designer. This limitation only allows creation of simplest jobs or a start-up of bigger jobs.
The above steps show the features of AWS Glue. Some of them could be omitted, if one would like to create his/her own way of connecting to a different data source using credentials stored in AWS Secrets Manager instead of creating Connection in AWS Glue. Additionally, there are a couple more useful functions of AWS Glue that were omitted in this article, like Workflow, or Triggers. Apart from the nice sides of AWS Glue, there are some disadvantages that need to be taken into consideration. Those will be mentioned in next article about AWS Glue.
AWS Glue is, amongst other AWS services, a great choice for a Big Data project.
Read more